Why Our Current Approach to AI is Fundamentally Flawed
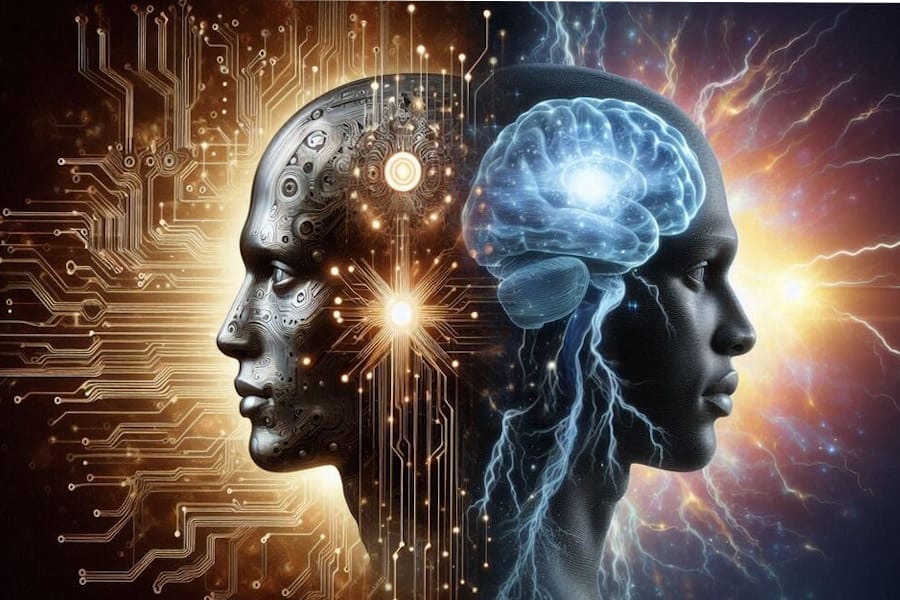
Publish Date: Last Updated: 10th February 2025
Author: nick smith - With the help of CHATGTP
Artificial intelligence has made tremendous leaps in recent years, offering us tools that were previously thought to exist only in science fiction. From virtual assistants to autonomous vehicles and complex data processing, the applications of AI seem boundless. However, as exciting as these advancements are, there are fundamental issues with our current approach to AI development. Chief among them is the excessive energy consumption of AI systems, the limitations of data training methodologies, and the absence of intuitive environmental awareness and adaptive learning mechanisms akin to the human brain.
The Energy Demand of Modern AI Systems
The power requirements of contemporary AI systems are astounding. While a single AI request from a user may seem harmless, the energy demand behind the scenes is significant. A study from the University of Massachusetts, Amherst, for instance, reported that training a single large AI model can emit over 626,000 pounds of CO₂, equivalent to the lifetime emissions of five average cars .
OpenAI’s GPT-3, one of the largest language models, is estimated to have used around 1,287 MWh (megawatt-hours) of energy during its training phase alone, with a carbon footprint comparable to the lifetime emissions of an average American . When we consider that the human brain consumes only about 20 watts of power to complete similar tasks—such as pattern recognition, language processing, and problem-solving—it becomes clear that AI lacks the efficiency we see in biological systems.
Understanding the Energy Efficiency of the Human Brain
The human brain achieves remarkable feats with a minimal energy budget. Its unique architecture, which evolved over millions of years, enables it to process complex stimuli while conserving energy. This disparity in energy consumption highlights a crucial difference: current AI models lack a way to emulate the brain's energy-efficient processes. The brain relies on its ability to perform complex, multi-modal learning in a highly dynamic, adaptable way, consuming less energy as repetitive tasks become automatic. For example, once the brain has mastered a task through learning, it is able to perform that task with minimal energy by relegating it to “automatic” pathways.
In contrast, AI systems typically consume the same amount of energy regardless of how often a task is repeated. This indicates that, despite incredible advancements, our approach to AI lacks a vital “adaptive efficiency” that would allow it to improve its performance and minimize energy use over time.
Why Current AI Training Models Are Incomplete
At the core of this inefficiency is the way we train AI. Current models rely on vast datasets composed of millions of images, texts, and other data to create an expansive, though sometimes shallow, knowledge base. This approach, while effective for training models to perform specific tasks, is not conducive to a true, holistic understanding of the world. Humans, on the other hand, learn from multi-sensory, real-world experiences.
Humans do not need to see countless images to recognize an object. For example, we understand what a “cat” is by seeing, touching, hearing, and interacting with one in 3D space. This rich, multi-dimensional interaction gives us an innate ability to recognize a cat from any angle, in any context, and even apply this knowledge to recognize other animals or objects in similar categories.
Creating AI That Mimics the Brain’s Architecture
For AI to reach a new level of efficiency and adaptability, it must evolve beyond current models toward a structure that emulates the human brain. The brain is organized into specialized regions—each dedicated to a specific sensory input or function, such as sight, hearing, or balance. These regions are interconnected, allowing for seamless communication and adaptive decision-making based on environmental context.
To achieve similar flexibility, future AI systems should be structured in a more modular way. Such systems could involve multiple dedicated processors or networks, each responsible for a particular “sense” or type of input, that would be able to share information dynamically. This approach would enable AI to process complex inputs more holistically rather than isolating them as separate tasks. Additionally, as in the human brain, AI would benefit from being able to “rewire” itself, adapting its own code to optimize performance and enhance learning over time.
The Role of Quantum Computing in AI’s Future
The human brain’s remarkable efficiency and possible quantum processing capabilities may offer insights into what AI needs to become truly self-aware. Theories in neuroscience propose that the brain may rely on quantum mechanics to enable certain cognitive functions, potentially explaining phenomena such as consciousness, memory, and sensory integration. Recent research on avian navigation supports the idea that biological systems can utilize quantum processing to interact with complex natural phenomena.
If AI is to achieve true awareness, a breakthrough in quantum computing may be necessary. Quantum processors offer the potential to perform complex calculations at a fraction of the energy required by traditional silicon-based processors, with quantum states allowing for unprecedented levels of efficiency and processing speed. As quantum computing technology advances and potentially becomes miniaturized, it could enable AI systems to process information with the speed and adaptability that resemble biological brains.
A quantum-enhanced AI could, in theory, recognize patterns and make decisions based on highly complex, interrelated data sets, similar to the way a human might instinctively recognize and respond to their surroundings. By combining quantum computing with an adaptive, modular architecture, we may come closer to developing AI that is not only vastly more energy-efficient but also more conscious of its environment and capable of evolving over time.
The Path Forward: An AI That Can Truly Understand
AI holds incredible promise, yet current approaches show the limitations of focusing on expansive data training over creating adaptive, efficient models that can “understand” rather than simply respond. As we seek to build AI that more closely mirrors the remarkable capabilities of the human brain, we will need to integrate multi-sensory inputs, self-adaptive programming, and potentially quantum processing.
A paradigm shift is required to design AI that is truly sustainable, intuitive, and conscious of its surroundings. Only then will we be able to create AI systems that, much like the human brain, achieve extraordinary feats with minimal energy while continuously learning and evolving in real-time.
Trending AI Articles
AI Questions and Answers section for Why Our Current Approach to AI is Fundamentally Flawed
Welcome to a new feature where you can interact with our AI called Jeannie. You can ask her anything relating to this article. If this feature is available, you should see a small genie lamp in the bottom right of the page. Click on the lamp to start a chat or view the following questions that Jeannie has answered relating to Why Our Current Approach to AI is Fundamentally Flawed.
Be the first to ask our Jeannie AI a question about this article
Look for the gold latern at the bottom right of your screen and click on it to enable Jeannie AI Chat.