What is Multimodal AI
Bridging Multiple Data Modalities in Artificial Intelligence
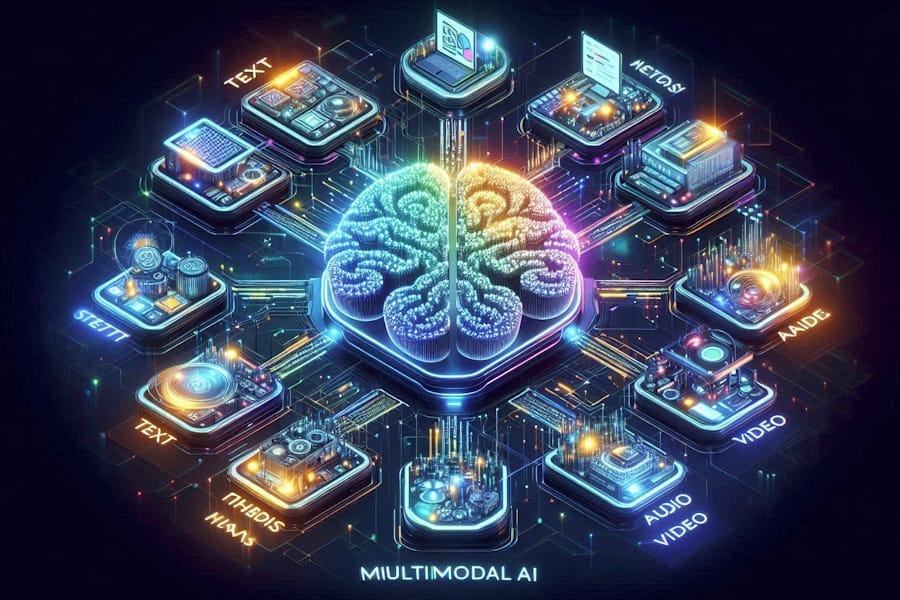
Publish Date: Last Updated: 3rd June 2025
Author: nick smith - With the help of CHATGTP
New - How to Build a Multimodal AI Model - Step-by-Step Tutorial for Beginners
Artificial Intelligence (AI) has undergone tremendous evolution in recent years, moving from specialized, single-task systems to versatile, integrated models capable of solving complex problems. A key concept driving this advancement is multimodality. Multimodality is a cornerstone in the development of AI that brings multiple types of data together for better, more robust decision-making. In this article, we will explore what multimodality means, how it works, its applications, and the implications it has for the future of artificial intelligence.
What is Multimodality?
Multimodality refers to the ability of a system—often an AI or machine learning model—to understand and process multiple types of data, or "modalities." These modalities can include text, images, audio, video, and more. Multimodal AI models are designed to integrate these various types of data simultaneously, allowing them to generate richer, more nuanced outputs.
In simple terms, multimodality allows AI to combine information from multiple sensory channels, much like how humans understand the world. For example, when we interact with our environment, we take in visual cues, sounds, text, touch, and smell, combining them to make sense of our surroundings. Similarly, a multimodal AI model is built to process multiple data sources in order to solve problems more effectively.
IT and computer science courses
How Does Multimodality Work?
Multimodal AI systems leverage deep learning and neural networks to combine data from different modalities. Here is how it generally works:
-
Data Acquisition: The AI collects information from multiple data sources. For instance, it could receive input from text, images, audio recordings, and video feeds.
-
Feature Extraction: Each type of data is processed using specialized algorithms that extract relevant features. For example:
- Images: Features such as shapes, colors, textures, and objects are extracted using convolutional neural networks (CNNs).
- Text: Features such as syntax, semantics, and entity relationships are identified using natural language processing (NLP) tools.
- Audio: Sound signals are processed to detect pitch, tone, and other acoustic features.
-
Fusion Layer: The extracted features are combined in a fusion layer, which integrates data from all the different modalities. This integration can be simple (such as concatenation) or involve more sophisticated methods (like attention mechanisms) that selectively weigh the importance of different features based on the task.
-
Joint Learning: Once the data is fused, the AI uses it to make predictions, perform classifications, or generate outputs. The key here is that the system learns a joint representation of the multimodal data, which allows it to produce better, context-aware decisions.
Applications of Multimodality
The potential applications of multimodal AI are vast, and many industries are already reaping the benefits of this approach. Below are some areas where multimodality is having a significant impact:
1. Healthcare
Multimodal AI is transforming healthcare by combining medical images, electronic health records, and genetic data to provide more accurate diagnoses. For example:
- In cancer diagnosis, a multimodal AI model can analyze radiological images, pathology slides, and patient records together, leading to better-informed decisions about the patient’s condition.
- AI-based diagnostic assistants can integrate text data from doctor's notes with visual data from medical scans to offer comprehensive patient assessments.
2. Virtual Assistants
Multimodal capabilities are crucial in virtual assistants like Amazon Alexa, Google Assistant, and Apple Siri, which combine voice, image, and text inputs. They interpret spoken commands, facial expressions, and contextual information to provide accurate responses. For instance, a smart assistant can read text on a product package, hear a user’s query, and visually analyze an item, providing a holistic response.
3. Autonomous Vehicles
Multimodal AI systems are at the core of autonomous driving technology. Self-driving cars rely on data from multiple sensors, such as cameras, LiDAR, radar, and GPS. The fusion of these inputs helps the vehicle understand its surroundings, detect obstacles, identify road signs, and navigate safely—all without human intervention.
4. Content Creation and Accessibility
Multimodal Transformers like OpenAI's GPT-4 are being used to create content that bridges different data types. These models can generate captions for images, write articles based on video content, or even summarize complex scenes in a movie—all of which enhance the accessibility of content for people with disabilities. For example, video captioning helps the hearing-impaired by turning spoken content into readable text, while image-to-text descriptions help the visually impaired.
5. Entertainment and Gaming
In video games and VR, multimodal AI can understand gestures, facial expressions, and voice commands to provide a more immersive experience. The game environment can adjust dynamically based on player emotions, making the gameplay more engaging and personalized.
Benefits of Multimodal AI
- Rich Context Understanding: By using multiple modalities, AI can understand more context, leading to better decision-making. For example, an AI chatbot that processes text and images can understand a customer’s needs more thoroughly.
- Reduced Ambiguity: Multimodal systems reduce ambiguity in decision-making. An image of a cat combined with the word "cat" allows the system to confirm its classification accurately.
- Improved Interaction: By combining audio, visual, and text inputs, multimodal systems are more interactive and capable of more human-like communication.
Challenges in Multimodality
While multimodality is incredibly promising, it’s not without its challenges:
-
Data Integration Complexity: Combining different types of data is complex because each modality may have different structures and forms of representation. For instance, images have spatial structures, while text is sequential. Designing models that can adequately learn from multiple types of input remains a significant challenge.
-
Data Quality and Availability: Multimodal AI requires large datasets of high-quality, labeled data from each modality. Collecting such data can be time-consuming and expensive. Furthermore, ensuring the data from each modality aligns correctly is critical to avoid learning inconsistencies.
-
Computational Cost: Multimodal models are computationally intensive. Integrating multiple data sources and learning from them requires significant computational power, often necessitating specialized hardware such as GPUs or TPUs.
The Future of Multimodal AI
The future of multimodal AI is incredibly promising. As AI becomes more advanced, the ability to handle and synthesize information from various modalities will enable more sophisticated interactions, contextual understanding, and decision-making capabilities. Here are some exciting possibilities:
-
Multimodal AI for Education: Personalized educational tools using multimodal AI can analyze text, voice, and video interactions to understand a student's strengths and weaknesses and adapt the teaching material accordingly.
-
Human-Robot Interaction: Robots of the future will be able to understand verbal commands, gestures, and environmental cues simultaneously, making them more adept at assisting in everyday tasks and interactions.
-
Cross-Lingual Multimodality: Advances are also being made in making multimodal systems capable of cross-lingual understanding. For example, a system could translate gestures, emotions, and spoken language, making cross-cultural communication more seamless.
MultiModal AI on YouTube
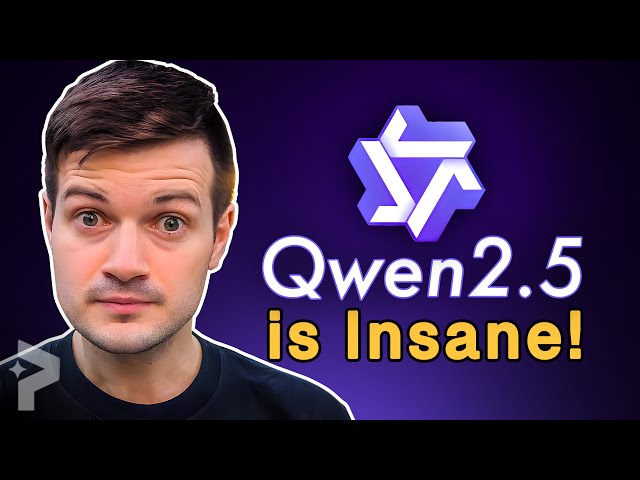
You can run this multimodal AI model on a laptop
YouTube Channel: Plivo
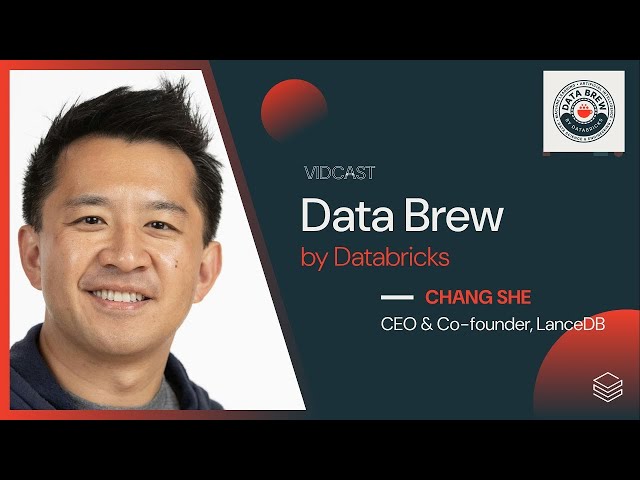
Multimodal AI & Next Gen Databases | Data Brew | Episode 42
YouTube Channel: Databricks
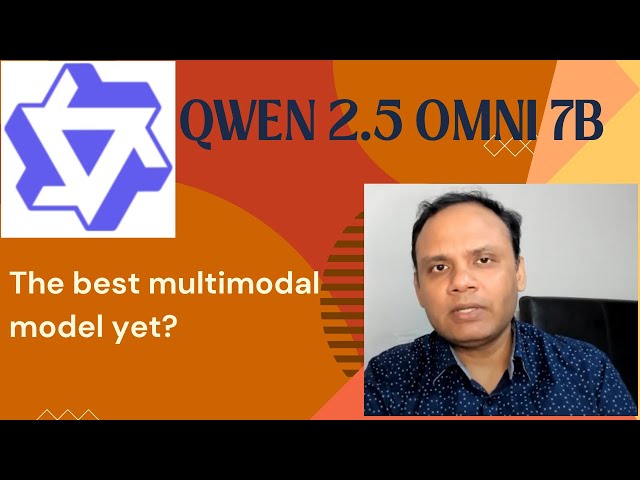
Qwen2.5 Omni 7B - The Best Multimodal AI Model Yet?
YouTube Channel: Bytes of AI
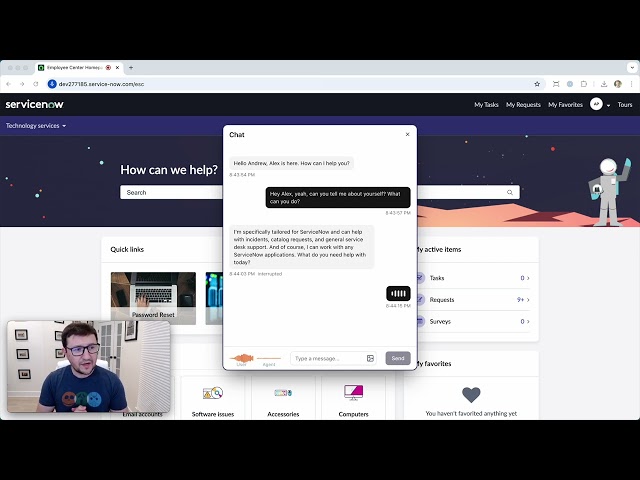
Customer Support Multimodal AI Agents in ServiceNow
YouTube Channel: Voice2Me
Conclusion
Multimodality is an exciting frontier in artificial intelligence that aims to replicate the way humans perceive and process information by fusing different data types. It’s a powerful tool that enhances AI’s understanding of complex, real-world scenarios and is already driving breakthroughs across healthcare, entertainment, autonomous vehicles, and more. Despite the challenges in data integration, computational power, and training complexity, the opportunities presented by multimodal AI are transformative.
As we advance toward building more integrated, intelligent systems, the combination of different modalities will undoubtedly play a crucial role in shaping the future of AI. Whether through enhancing user interactions or making medical diagnoses more accurate, multimodality is set to push the boundaries of what artificial intelligence can achieve.
Recent AI Articles
AI Questions and Answers section for Understanding Multimodal AI: Bridging Multiple Data Modalities in Artificial Intelligence
Welcome to a new feature where you can interact with our AI called Jeannie. You can ask her anything relating to this article. If this feature is available, you should see a small genie lamp in the bottom right of the page. Click on the lamp to start a chat or view the following questions that Jeannie has answered relating to Understanding Multimodal AI: Bridging Multiple Data Modalities in Artificial Intelligence.
Visitor asked: In ealth care how can multimodal help
Jeannie’s answer to the question: Multimodal AI can significantly transform the health care sector in numerous ways. Firstly, it can aggregate and interpret data from various sources like medical records, imaging data, genetic information, and patient biometrics to provide a holistic view of a patient's health. This can facilitate personalized treatment plans, predictive analyses, and early diagnosis. Moreover, the consolidation and analysis of multimodal data can enhance telemedicine services, deliver more accurate remote monitoring, and generate advanced warnings for deteriorating patients which could minimize emergency hospitalizations. Lastly, it can improve the interpretation of radiology images, CT scans, and MRIs which can aid in early detection of diseases such as cancer. Therefore, the potential of Multimodal AI in the health care sector is immense, promising to revolutionize patient care and health outcomes.
Date: 2025-02-10