How to Build a Multimodal AI Model
Step-by-Step Tutorial for Beginners
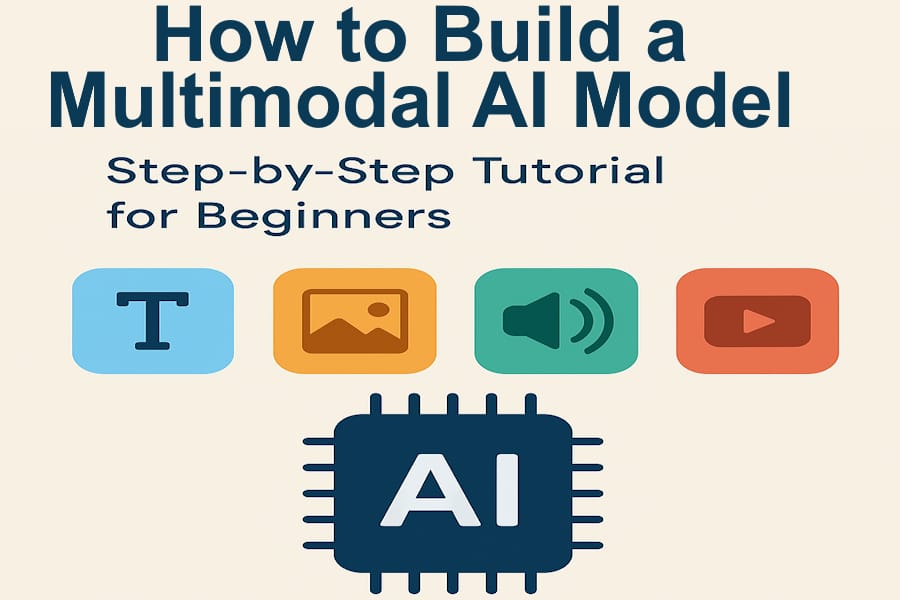
Publish Date: Last Updated: 25th April 2025
Author: nick smith - With the help of CHATGTP
Introduction
In today’s AI world, it’s no longer enough for machines to just read or see—they need to do both (and more). That’s where Multimodal AI comes in.
Multimodal AI refers to models that can understand and generate content from multiple types of data—like text, images, audio, and even video—at the same time. Think of it as building an AI that can not only read your message but also see the photo you attached and respond appropriately. View our article on What is MultiModal
In this tutorial, you’ll learn:
✅ What Multimodal AI is
✅ Real-world examples of where it’s used
✅ How to build a simple Multimodal AI model using Python
✅ What tools and datasets you need
✅ How to run it all on Google Colab (free!)
✅ How to apply it in areas like education, healthcare, and content creation
💡 No deep AI background? No problem. This guide is designed to be beginner-friendly and easy to follow.
🗂️ Table of Contents
- What is Multimodal AI?
- Tools You’ll Use
- Hands-On: Building a Multimodal Model
- Beyond Basics: Fine-Tuning or Customizing
- Use Cases
- FAQ
- Conclusion
🧩 What Is Multimodal AI?
Multimodal AI is a type of artificial intelligence that can understand and generate information from more than one kind of input, also known as modalities.
The most common modalities are:
- 📝 Text – written language (like this sentence).
- 🖼️ Images – photos, drawings, screenshots.
- 🔊 Audio – speech, music, sound effects.
- 🎥 Video – sequences of images with audio and motion.
- 👁️🗨️ Sensor data – from devices like self-driving cars or smartwatches.
Traditional AI models are “unimodal”—they only handle one type of data. For example:
- A text-based chatbot like GPT-3.
- An image classifier that labels photos.
- A speech-to-text app like Whisper.
But Multimodal AI can take a combination of inputs—like an image and a question—and respond intelligently based on both.
🚀 Real-World Examples of Multimodal AI
Here’s where you’ve already seen it in action:
Use Case | Description |
---|---|
🖼️ Image Captioning | AI looks at an image and writes a description: “A dog jumping in the grass” |
❓ Visual Question Answering | You ask: “What color is the cat?” and the AI looks at the image to answer “gray” |
🔍 Image + Text Search | Type “red shoes” and AI finds matching images across the web or in a database |
📹 Video Content Analysis | AI breaks down video scenes, identifies faces, actions, or even sentiment |
🤖 Famous Models That Use Multimodal Learning
- CLIP (OpenAI): Connects images and text for powerful search and understanding.
- DALL·E: Generates images from text prompts.
- GPT-4V (Vision): Understands images and combines them with text responses.
- Google Gemini: Google's multimodal LLM designed to handle a mix of data inputs.
💡 Why Is It So Important?
Multimodal AI makes machines more human-like in how they process information. Humans naturally combine what we see, hear, and read to understand the world—and now AI can too.
This opens doors to better:
- Virtual assistants.
- Educational tools.
- Accessibility tech (e.g., for the visually impaired).
- Smart search engines.
- Creative design tools.
🛠️ Tools and Technologies You’ll Use
To build your first Multimodal AI model, you don’t need a powerful computer or expensive software. Everything can be done using free tools and cloud platforms like Google Colab.
Here’s what you’ll use:
🐍 Python
Python is the go-to language for AI and machine learning. It's readable, flexible, and supported by tons of libraries we’ll use throughout this tutorial.
🤗 Hugging Face Transformers
This is one of the most popular libraries for loading pretrained models—like CLIP, BLIP, and other multimodal architectures.
Command (bash)
pip install transformers
🔥 PyTorch
PyTorch is a deep learning framework that makes it easy to train and run neural networks. It’s what many models under the hood (like CLIP) use.
Command (bash)
pip install torch torchvision
📝 Tip: If you're using TensorFlow instead, you can still follow along conceptually.
🖼️ PIL / OpenCV
These are image processing libraries. You’ll use them to load and manipulate image files before passing them into your AI model.
Command (bash)
pip install pillow
🌍 Google Colab
Google Colab is a free online environment where you can write and run Python code in the cloud—with access to GPUs!
- No installs required.
- You can share links to your code.
- Works right in your browser.
📚 Datasets
We’ll start with simple examples using built-in or small demo datasets, but if you want to go further, check out:
- COCO (Common Objects in Context): Text + image data.
- Flickr8k/30k: Images with captions.
- VQA Dataset: Visual question answering pairs.
🔄 Optional APIs If you want to explore advanced models (like GPT-4 with vision), you can use APIs like:
- OpenAI’s API (for GPT-4V).
- Google Gemini API (if publicly available).
These usually require API keys and may not be free, so we’ll focus on fully open tools for the core tutorial.
🧪 Hands-On: Building a Multimodal Model with CLIP
In this part of the tutorial, you’ll:
- Load a pre-trained multimodal model (CLIP).
- Feed it an image and a few text prompts.
- See how it ranks which caption best matches the image.
We’ll be using Google Colab, so you won’t need to install anything locally.
✅ Step 1: Open a Colab Notebook
Open this starter notebook on Google Colab
🧰 Step 2: Install Dependencies
At the top of your notebook, run this:
!pip install torch torchvision torchaudio
!pip install transformers
!pip install pillow
🖼️ Step 3: Load an Image and Some Captions
Upload an image or use one from the internet. Then write a few captions that the model can choose between.
from PIL import Image
import requests
from io import BytesIO
# Load an example image
url = "https://images.unsplash.com/photo-1601758123927-1967f6105551"
image = Image.open(BytesIO(requests.get(url).content))
image.show()
#Example captions
texts = ["A dog playing in the grass", "A cat sleeping on a bed", "A man riding a bicycle"]
🧠 Step 4: Load CLIP Model
Use Hugging Face Transformers to load CLIP.
from transformers import CLIPProcessor, CLIPModel
model = CLIPModel.from_pretrained("openai/clip-vit-base-patch32")
processor = CLIPProcessor.from_pretrained("openai/clip-vit-base-patch32")
🧪 Step 5: Run the Model
Encode the image and text, then compute similarity scores.
inputs = processor(text=texts, images=image, return_tensors="pt", padding=True)
outputs = model(**inputs)
# Similarity score between image and each caption
import torch
logits_per_image = outputs.logits_per_image # shape: [1, num_captions]
probs = logits_per_image.softmax(dim=1)
# Show results
for i, text in enumerate(texts):
print(f"{text}: {probs[0][i].item():.4f}")
📊 Example Output
A dog playing in the grass: 0.9123
A cat sleeping on a bed: 0.0412
A man riding a bicycle: 0.0465
The model thinks the image is most likely of a dog playing in the grass—and it's right!
Beyond Basics: Fine-Tuning or Customizing Your Own Multimodal Model
Once you’ve experimented with a pre-trained model, you might want to adapt it to your specific use case. For example:
- Product-specific image captioning (e.g., fashion items).
- Matching photos with blog content.
- Creating a custom visual Q&A bot.
Fine-tuning lets you do that.
🚨 Note Before You Start
Fine-tuning requires:
- A GPU (Colab Pro or a local machine with CUDA).
- A paired dataset (images + text, like captions or descriptions).
- More code and compute than basic inference.
We’ll use the BLIP model for this example (it’s made for tasks like captioning and VQA).
✅ Step 1: Install Required Libraries
!pip install transformers datasets torchvision
🖼️ Step 2: Prepare Your Dataset
You need image-text pairs. You can:
- Use a public dataset like Flickr8k.
- Or create your own dataset in this format:
{
"image_path": "path/to/image1.jpg",
"caption": "A cat sitting on a sofa."
}
For custom datasets, save them as a .csv
or .json
file and load them using datasets
or plain Python.
📦 Step 3: Load BLIP Model
from transformers import BlipProcessor, BlipForConditionalGeneration
processor = BlipProcessor.from_pretrained("Salesforce/blip-image-captioning-base")
model = BlipForConditionalGeneration.from_pretrained("Salesforce/blip-image-captioning-base")
🧠 Step 4: Create a Training Loop (Simplified Example)
from datasets import load_dataset
from torch.utils.data import DataLoader
import torch
# Assuming you have a dataset with 'image' and 'caption' columns
dataset = load_dataset("your_dataset_script_or_path")
def preprocess(example):
image = Image.open(example["image_path"]).convert("RGB")
inputs = processor(images=image, text=example["caption"], return_tensors="pt", padding=True)
return inputs
train_loader = DataLoader(dataset["train"], batch_size=4, shuffle=True)
optimizer = torch.optim.AdamW(model.parameters(), lr=5e-5)
model.train()
for batch in train_loader:
outputs = model(**batch, labels=batch["input_ids"])
loss = outputs.loss
loss.backward()
optimizer.step()
optimizer.zero_grad()
print("Loss:", loss.item())
🔄 This is a simplified loop. In practice, use Trainer
from Hugging Face or a training script template for stability and logging.
🧪 Test Your Fine-Tuned Model
Inference after training
image = Image.open("path/to/test_image.jpg").convert("RGB")
inputs = processor(images=image, return_tensors="pt")
out = model.generate(**inputs)
print(processor.decode(out[0], skip_special_tokens=True))
💡 Tip: Use Trainer API for Cleaner Training
If you prefer Hugging Face’s built-in training utilities, wrap your data and model using Trainer
, TrainingArguments
, etc.
🌍 Real-World Applications of Multimodal AI
Multimodal AI isn’t just a cool research concept—it’s powering real innovations in the apps, tools, and platforms we use every day. Here are some of the most exciting and impactful use cases:
🧑🏫 1. Education & e-Learning
Multimodal AI helps create smart, interactive learning experiences. For example:
- Generate visual explanations from text-based concepts.
- Answer questions based on diagrams or charts.
- Power virtual tutors that can read a student’s question and the attached worksheet.
💡 Imagine asking your AI assistant: “What does this graph mean?”—and it explains it back in plain English.
🩺 2. Healthcare & Diagnostics
Multimodal AI is being used to combine medical imaging with clinical notes for better diagnosis.
Examples:
- Detecting conditions from X-rays with supporting text.
- Generating patient summaries that merge lab reports with visuals.
- AI-assisted ultrasound interpretation.
🧠 It sees the scan + reads the symptoms = smarter, faster analysis.
🛍️ 3. E-Commerce & Retail
AI that understands product images and descriptions can:
- Auto-generate product captions.
- Recommend similar products by “visual + textual” similarity.
- Improve search results using visual cues.
🛒 Type “green running shoes” and get smart results across the store catalog.
♿ 4. Accessibility Tools
Multimodal AI can help users with disabilities by:
- Describing images for the visually impaired.
- Translating sign language from video into text.
- Converting complex data visuals into spoken summaries.
🔊 “There is a person in the image holding a cup of coffee.”
🎥 5. Content Creation & Social Media
Creators are using AI that understands both visuals and words to:
- Auto-caption videos and photos.
- Create content summaries.
- Match background music with scene mood.
🎨 Generate thumbnails, hashtags, and even script suggestions based on your video.
🔍 6. Smart Search Engines
Modern search goes beyond keywords. Multimodal AI powers:
- Visual search (“search by image”).
- Text+image hybrid queries.
- Semantic search that understands content meaning.
📸 Drag an image into the search box and type “similar but red”—the AI gets it.
🚀 Bonus: Other Cool Applications
- Multimodal chatbots that respond to images, text, and even voice.
- Autonomous vehicles that process video, lidar, and voice commands.
- Multimodal emotion recognition from facial expression + voice tone + text.
❓ Frequently Asked Questions (FAQ)
🤔 What is Multimodal AI?
Multimodal AI is artificial intelligence that can understand and process multiple types of data at once, such as images, text, audio, and video. Unlike traditional models that only handle one type (e.g., text-only chatbots), multimodal models can combine different types of inputs for deeper understanding and better output.
🧠 What’s an example of a multimodal AI model?
Popular examples include:
- CLIP: Connects images with text for powerful matching and search.
- DALL·E: Generates images from text prompts.
- BLIP: Creates image captions or answers questions about images.
- GPT-4V: Can “see” images and generate smart responses using them.
🔍 Why is Multimodal AI better than Unimodal AI?
Because it mimics how humans think—we use multiple senses at once (sight, sound, language). Multimodal AI can:
- Understand context better.
- Generate more accurate responses.
- Solve complex tasks like visual Q&A or image generation from text.
💻 Do I need a powerful computer to run this?
Not at all! You can use Google Colab, which is free and runs everything in the cloud—even with GPU access for faster performance.
🧪 Can I train my own multimodal model?
Yes! In this tutorial, we show how to fine-tune a model like BLIP using your own image-caption data. It requires:
- A GPU (Colab Pro or a local machine).
- A dataset of image-text pairs.
- Basic knowledge of Python and PyTorch.
📚 Where can I get free multimodal datasets?
Some great places to start:
- Flickr8k.
- COCO Captions.
- VQA Dataset.
- LAION (huge dataset used to train CLIP).
🤖 Can I use GPT-4 with images?
Yes, if you have API access to GPT-4 with vision (GPT-4V). It allows you to input images and receive intelligent text-based responses. It's part of OpenAI’s ChatGPT Plus plan or available via API.
🧠 What programming skills do I need?
You’ll need:
- Basic Python.
- Familiarity with Jupyter or Colab notebooks.
- Some experience with packages like
transformers
,torch
, ordatasets
.
But don’t worry—this tutorial is beginner-friendly and walks you through everything step-by-step.
🎉 Conclusion: What You Can Build Next
You’ve just taken your first steps into the world of Multimodal AI—congrats!
By now, you’ve learned: ✅ What Multimodal AI is and why it matters
✅ How to use pre-trained models like CLIP
✅ How to build a working prototype that understands images and text
✅ How to fine-tune a multimodal model on your own dataset
✅ Real-world applications and inspiration for future projects
🚀 Where to Go from Here
Now that you've got the basics, here are a few ideas to keep exploring:
🔹 Build an image captioning tool for your own photos
🔹 Train a product recommendation system using both image and text metadata
🔹 Create an AI assistant that answers questions about uploaded charts, infographics, or diagrams
🔹 Start a personal AI research project using datasets like COCO or VQA
🔹 Try GPT-4V or Google Gemini if you have access, and explore advanced vision-language tasks
🙌 Help Others Learn
If you found this guide helpful:
- Share it with your peers or learning community 💬.
- Star the GitHub repo (if you host this project).
- Drop us a comment or suggestion for improvement ✍️.
💡 Final Thought
Multimodal AI isn’t just the future—it’s already here. From creative tools and virtual assistants to smarter search engines and accessible tech, the possibilities are endless.
You now have the knowledge and tools to start building your own intelligent systems that understand the world more like we do—through sight, language, sound, and context combined.
Keep experimenting. Keep learning. The frontier of AI is multimodal. 🌐🤖🔥
More Actionable AI Articles
AI Questions and Answers section for How to Build a Multimodal AI Model: Step-by-Step Tutorial for Beginners
Welcome to a new feature where you can interact with our AI called Jeannie. You can ask her anything relating to this article. If this feature is available, you should see a small genie lamp in the bottom right of the page. Click on the lamp to start a chat or view the following questions that Jeannie has answered relating to How to Build a Multimodal AI Model: Step-by-Step Tutorial for Beginners.
Be the first to ask our Jeannie AI a question about this article
Look for the gold latern at the bottom right of your screen and click on it to enable Jeannie AI Chat.