What Are AI Tokens?
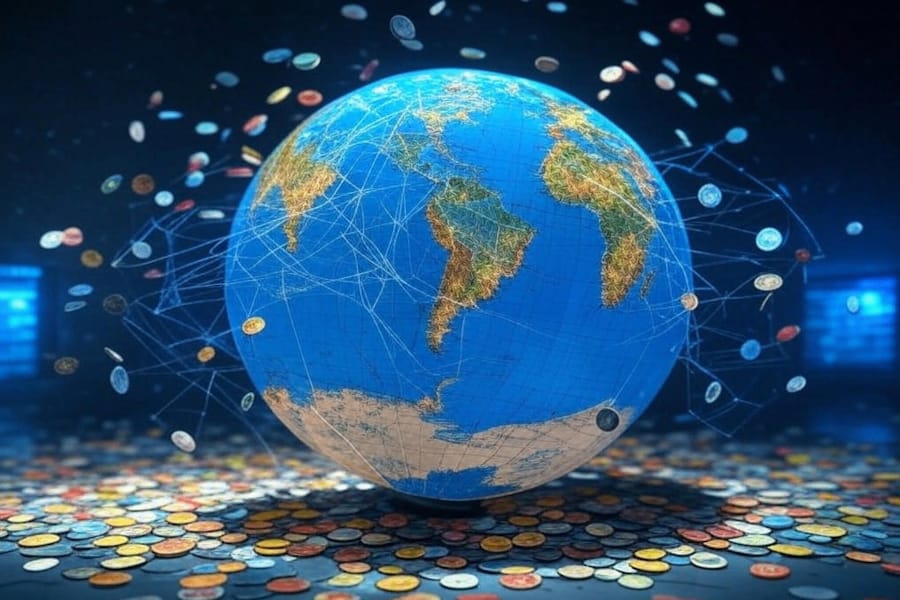
Publish Date: Last Updated: 30th June 2025
Author: nick smith - With the help of GROK3
An AI token is a unit of data used by AI models to process and generate human-like text, images, or other forms of output. In the context of NLP, a token typically represents a word, part of a word, or a punctuation mark, but it can also encompass more complex structures like phrases or symbols depending on the model’s design. Tokens are the building blocks that AI systems, such as large language models like GPT or Llama, use to interpret input data and produce meaningful responses.
For example, in the sentence “AI is transforming the world,” each word (“AI,” “is,” “transforming,” “the,” “world”) and the punctuation mark might be considered individual tokens. However, some models break words into smaller units, such as subwords or characters, to handle complex or rare words efficiently.
Why Are They Called Tokens?
The term token originates from linguistics and computer science, where it refers to a discrete unit of meaning or data. In AI, tokens are aptly named because they serve as standardized, manageable pieces of information that the model can process. Much like tokens in a board game represent distinct entities, AI tokens represent distinct pieces of language or data, enabling the model to analyze and manipulate them systematically.
How Do AI Tokens Work?
The process of working with AI tokens involves several key steps, collectively known as tokenization, which is the foundation of how AI models handle input and output data. Let’s break it down:
1. Tokenization: Breaking Down Input
Tokenization is the process of converting raw input data—such as text, code, or even images—into a sequence of tokens. This step is crucial because AI models cannot directly understand human language or raw data. Instead, they rely on numerical representations of tokens to perform computations.
- Word-Based Tokenization: Splits text into words or punctuation marks. For example, “Hello, world!” might become [“Hello,” “world,” “!”].
- Subword Tokenization: Breaks words into smaller units, often used in models like BERT or GPT. For instance, “unhappiness” might be tokenized as [“un,” “hap,” “piness”].
- Character-Based Tokenization: Treats each character as a token, useful for languages with complex scripts or code.
Popular tokenization algorithms, such as Byte Pair Encoding (BPE) or WordPiece, balance vocabulary size and flexibility, ensuring models can handle diverse inputs, including rare words or misspellings.
IT and computer science courses
2. Encoding Tokens into Numerical Data
Once tokenized, each token is mapped to a unique numerical identifier based on the model’s vocabulary. For example, the token “AI” might correspond to the number 500 in a model’s dictionary. This numerical representation allows the AI to process tokens mathematically.
3. Processing Tokens in the Context Window
AI models operate within a context window, which defines the maximum number of tokens they can process at once. For instance, a model with a context window of 4,096 tokens can analyze or generate text up to that limit in a single pass. The context window is critical because it determines how much information the model can “remember” when generating responses or making predictions.
During processing, tokens are fed into the model’s neural network, which uses attention mechanisms to weigh the relationships between tokens. This enables the model to understand context, grammar, and semantics, producing coherent and relevant outputs.
4. Generating Output
After processing the input tokens, the model generates output tokens, which are then decoded back into human-readable form. For example, a sequence of numerical token IDs is converted back into words or sentences, such as “AI is transforming the world” being generated as a response.
Why Are AI Tokens Important?
AI tokens are the linchpin of modern AI systems, and their significance can be understood through several lenses:
1. Enabling Natural Language Understanding
Tokens allow AI models to break down complex human language into manageable units, facilitating natural language understanding (NLU). By representing words, phrases, or symbols as tokens, models can analyze syntax, semantics, and context, enabling applications like chatbots, translation tools, and sentiment analysis.
2. Optimizing Computational Efficiency
Tokenization enhances computational efficiency by reducing the complexity of raw data. Instead of processing entire sentences or paragraphs as monolithic entities, models handle discrete tokens, which streamlines calculations and reduces memory usage. Efficient tokenization also allows models to scale to larger datasets and more complex tasks.
3. Supporting Scalability of Language Models
The design of tokens directly impacts the scalability of language models. Subword tokenization, for instance, enables models to handle vast vocabularies without requiring excessive memory. This is particularly important for multilingual models that must process diverse languages and scripts.
4. Defining Model Capabilities
The number of tokens a model can process (its context window) defines its ability to handle long-form content or maintain coherence in extended conversations. Larger context windows, made possible by advances in token processing, allow models to tackle tasks like summarizing lengthy documents or generating detailed narratives.
5. Driving Innovation in AI Applications
Tokens are not limited to text-based AI. In multimodal models, tokens represent diverse data types, such as pixels in images or audio waveforms. This versatility fuels innovation in fields like computer vision, speech recognition, and generative AI, where tokens bridge different modalities.
Challenges and Considerations with AI Tokens
While tokens are indispensable, they come with challenges that researchers and developers must address:
- Token Limitations: Models with smaller context windows struggle with long texts, leading to truncated or incomplete processing.
- Tokenization Bias: The choice of tokenization method can introduce biases, such as poor handling of certain languages or dialects.
- Computational Costs: Processing large numbers of tokens requires significant computational resources, impacting energy consumption and accessibility.
- Token Efficiency: Some tokenization methods produce longer sequences than necessary, slowing down processing or increasing costs in API-based systems.
Ongoing research aims to address these issues by developing more efficient tokenization algorithms, expanding context windows, and optimizing hardware for token processing.
The Future of AI Tokens
As AI continues to advance, the role of tokens will only grow in importance. Emerging trends suggest several exciting developments:
- Dynamic Tokenization: Future models may adapt tokenization strategies on the fly, optimizing for specific tasks or languages.
- Token Compression: Techniques to reduce token counts without losing information could enhance efficiency and lower costs.
- Multimodal Tokens: As AI integrates text, images, and other data types, tokens will evolve to represent increasingly complex information.
- Ethical Token Design: Addressing biases in tokenization will be critical to ensuring equitable AI systems that serve diverse global populations.
AI Tokens Explained from YouTube
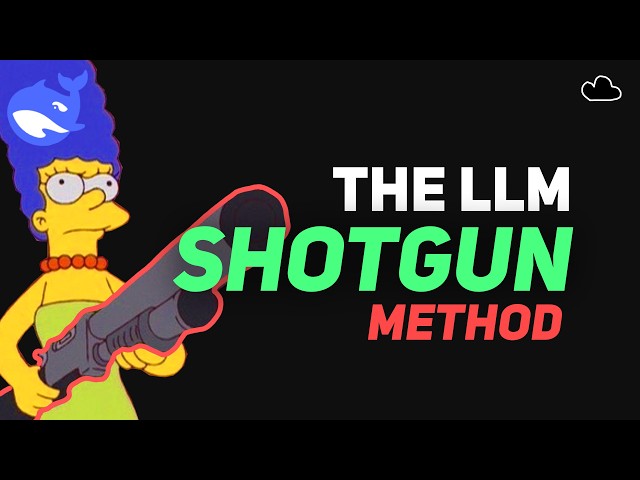
Why would anyone let LLMs predict 4 tokens at once?
YouTube Channel: bycloud
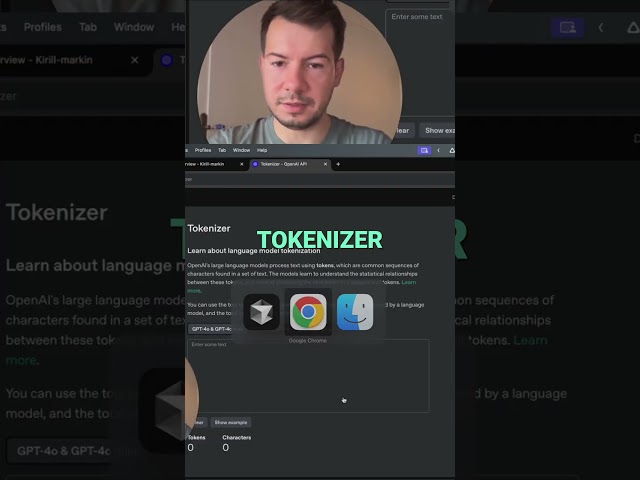
AI Tokens vs Letters: How AI 'Sees' Text
YouTube Channel: Kirill Markin
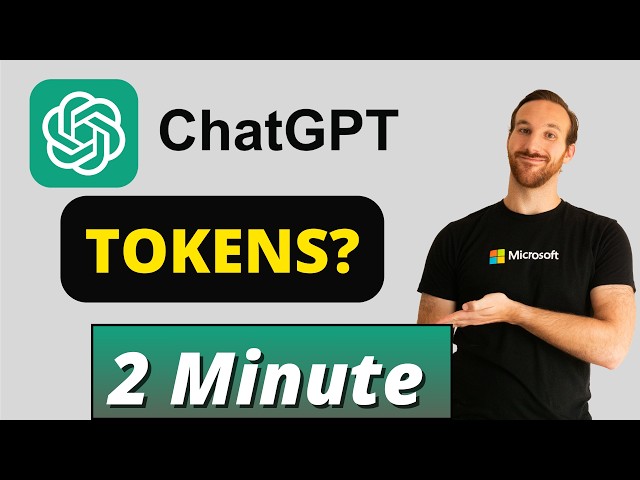
What is an AI Token? | LLM Tokens explained in 2 minutes!
YouTube Channel: Azure Innovation Station
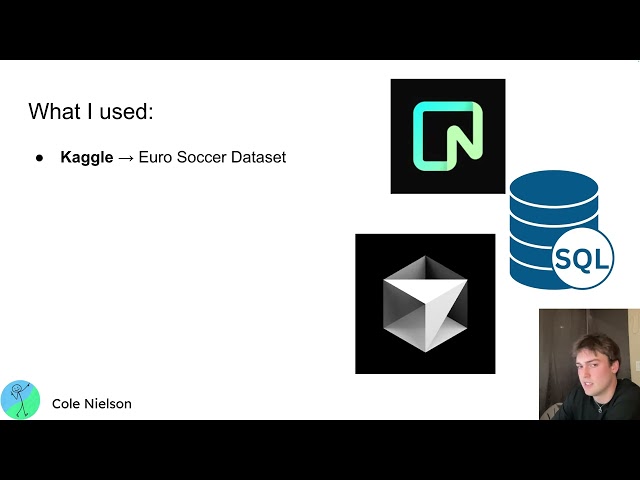
Using AI to query 33 Million token data set
YouTube Channel: Cole Nielson
Conclusion
AI tokens are the unsung heroes of artificial intelligence, enabling machines to understand and generate human-like outputs with remarkable precision. Through tokenization, tokens transform raw data into a format that AI models can process, driving natural language understanding and computational efficiency. Their role in defining the context window and supporting scalable language models underscores their importance in powering applications that shape our daily lives.
By appreciating the mechanics and significance of AI tokens, we gain insight into the inner workings of AI systems and their potential to revolutionize industries, from healthcare to education to entertainment. As research continues to refine tokenization techniques and expand their applications, tokens will remain at the heart of AI’s transformative journey.
Trending Articles
AI Questions and Answers section for What Are AI Tokens?
Welcome to a new feature where you can interact with our AI called Jeannie. You can ask her anything relating to this article. If this feature is available, you should see a small genie lamp in the bottom right of the page. Click on the lamp to start a chat or view the following questions that Jeannie has answered relating to What Are AI Tokens?.
Be the first to ask our Jeannie AI a question about this article
Look for the gold latern at the bottom right of your screen and click on it to enable Jeannie AI Chat.