The Evolution of Artificial Intelligence
From Concept to Cutting-Edge Technology
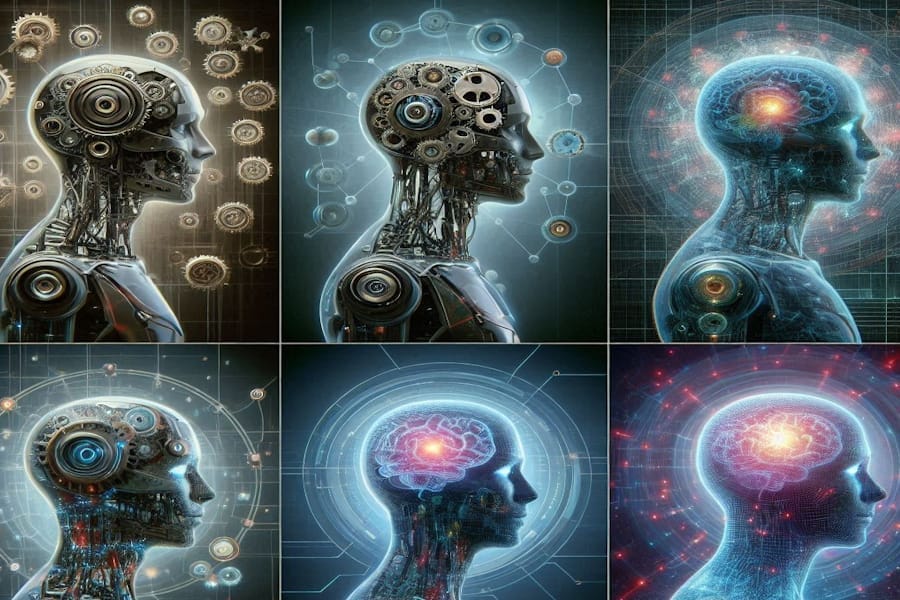
Publish Date: Last Updated: 10th February 2025
Author: nick smith - With the help of CHATGTP
Artificial intelligence (AI) has gone from an ambitious concept to a transformative force reshaping numerous fields. Its development has been driven by key milestones, from early theoretical foundations and pioneering machine learning techniques to the sophisticated deep learning systems of today. Let’s explore the historical evolution, technical advancements, and ethical challenges that have defined the journey of AI.
1. The Birth of AI: Early Concepts and Foundations
The concept of artificial intelligence predates computers, with ancient civilizations speculating about mechanical beings capable of human thought and action. However, AI’s formal beginnings can be traced back to the 20th century. Alan Turing, often called the father of computer science, introduced the Turing Test in 1950 as a benchmark for machine intelligence, challenging the notion of whether machines could think. This was soon followed by John McCarthy's 1956 workshop at Dartmouth College, where he coined the term "artificial intelligence" and laid the groundwork for AI research.
In the following decades, researchers focused on rule-based systems, which operated through predefined sets of instructions. While useful in limited contexts, these early AI models lacked the ability to learn from new data, marking a significant limitation
2. The Rise of Machine Learning: Data-Driven Intelligence
The 1980s and 1990s saw the emergence of machine learning (ML), a paradigm shift allowing AI to learn from data instead of following rigid instructions. Machine learning algorithms could now identify patterns, make decisions, and improve over time, propelling AI into practical applications. During this time, the development of artificial neural networks, inspired by the human brain's structure, laid the groundwork for more sophisticated models.
Statistical methods, such as decision trees and support vector machines, also gained traction, expanding the use cases for AI in areas such as finance, medicine, and manufacturing. However, the lack of sufficient computational power and large datasets limited the potential of these early machine learning models
3. The Deep Learning Revolution: Breakthroughs in Neural Networks
The 2010s marked a breakthrough for AI with the advent of deep learning, a subfield of machine learning that leverages multi-layered neural networks. Driven by access to massive datasets and advancements in computing power, deep learning achieved unprecedented success in tasks like image and speech recognition. AlexNet, a deep learning model, won the 2012 ImageNet competition, showcasing the power of neural networks and sparking widespread adoption of deep learning techniques.
Deep learning has enabled applications that were once thought impossible, from self-driving cars to real-time language translation and complex pattern recognition. Today, deep learning models like GPT-4 and BERT are powering natural language processing (NLP) systems, allowing machines to understand and generate human-like language
AI’s reach has expanded into nearly every industry, revolutionizing processes and enhancing decision-making. In healthcare, AI aids in early diagnosis and personalized treatment plans by analyzing large amounts of patient data. In finance, algorithms predict stock market trends and detect fraudulent transactions. Additionally, in transportation, AI powers autonomous vehicles, reshaping urban mobility and logistics.
The recent rise of AI-powered assistants, such as Siri and Alexa, has brought AI into everyday life, enhancing productivity and convenience. These applications are powered by complex algorithms that continue to learn from user interactions, becoming more accurate and efficient over time
5. Ethical Considerations and the Future of AI
As AI technologies become more powerful, ethical considerations have come to the forefront. Issues like bias, privacy, and job displacement are major concerns, with experts advocating for responsible AI development. The potential for AI to reinforce social biases, for instance, has raised concerns over fair treatment in hiring processes, loan approvals, and law enforcement.
Regulatory frameworks are emerging worldwide to address these issues. In the EU, the General Data Protection Regulation (GDPR) and the proposed AI Act aim to safeguard individual privacy and set ethical standards. As AI becomes increasingly integrated into society, creating transparent and accountable AI systems remains essential to mitigate risks and ensure public trust.
6. The Road Ahead: Emerging Trends and Challenges
The next frontier of AI involves advancements in reinforcement learning, quantum computing, and explainable AI. Reinforcement learning, where agents learn by interacting with environments and receiving feedback, is expected to improve robotics and autonomous systems. Quantum computing, though still in its infancy, holds the potential to solve complex problems beyond the capacity of classical computers, accelerating advancements in AI.
Moreover, explainable AI is gaining attention as organizations demand greater transparency. These models offer insights into decision-making processes, enabling users to understand how and why certain outcomes are reached.
The future of AI looks promising, with continuous advancements driving innovation across sectors. However, responsible development and regulation will be crucial in ensuring that AI remains a positive force for humanity.
Conclusion
AI’s journey from theoretical exploration to practical application has transformed it into one of the most influential technologies of our time. As we continue to push the boundaries, balancing innovation with ethics will be essential to harness AI’s full potential. The future of AI is likely to redefine industries, enhance quality of life, and unlock solutions to some of the world’s biggest challenges.
Trending AI Articles
AI Questions and Answers section for The Evolution of Artificial Intelligence: From Concept to Cutting-Edge Technology
Welcome to a new feature where you can interact with our AI called Jeannie. You can ask her anything relating to this article. If this feature is available, you should see a small genie lamp in the bottom right of the page. Click on the lamp to start a chat or view the following questions that Jeannie has answered relating to The Evolution of Artificial Intelligence: From Concept to Cutting-Edge Technology.
Be the first to ask our Jeannie AI a question about this article
Look for the gold latern at the bottom right of your screen and click on it to enable Jeannie AI Chat.