What are AI Weights?
The Backbone of Machine Learning Models
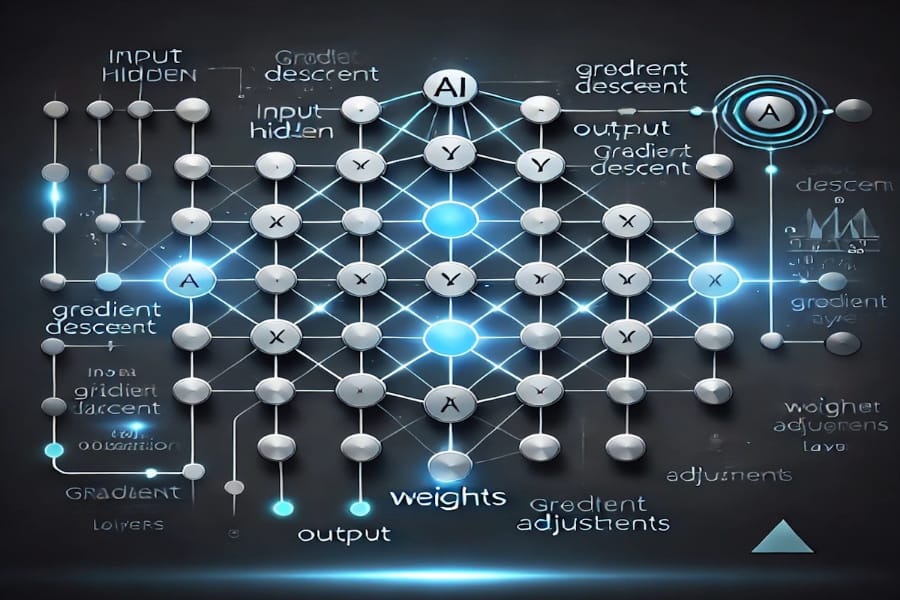
Publish Date: Last Updated: 5th June 2025
Author: nick smith - With the help of CHATGTP
Introduction to AI Weights
AI weights are fundamental components in artificial intelligence and machine learning models. They determine how much influence each input feature has on the model’s final decision. Understanding AI weights is crucial to optimizing machine learning models and improving their accuracy.
What Are AI Weights?
AI weights are numerical values assigned to the connections between nodes in artificial neural networks. They adjust how much each input contributes to the output. These values are refined through training, allowing the model to learn patterns in data and make accurate predictions.
In a simple neural network, weights work in conjunction with biases to transform input data through multiple layers, ultimately leading to an output. Mathematically, weights are part of the linear equation:
During training, these weights are optimized to reduce error and enhance the performance of the model.
How Weights Affect the Model Outcome
-
Prediction Accuracy: The correct adjustment of weights ensures that the model can generalize well to unseen data. Poorly tuned weights lead to overfitting or underfitting.
-
Feature Importance: Higher weights indicate that a feature significantly impacts the model’s output, whereas lower weights suggest minimal influence.
-
Neural Network Activation: Weights influence how neurons activate within hidden layers, ultimately affecting decision-making processes.
-
Model Complexity: Excessive weight values can lead to instability, making models prone to erratic predictions.
-
Optimization Efficiency: Properly initialized weights help models converge faster during training, improving learning speed and efficiency.
How AI Weights Are Adjusted
Weights are fine-tuned through an optimization process using algorithms such as gradient descent. The key steps include:
-
Forward Propagation: Inputs are multiplied by weights and passed through the neural network.
-
Loss Calculation: The error between predicted and actual outputs is measured using a loss function.
-
Backpropagation: The model calculates the gradient of the loss function concerning each weight.
-
Weight Update: Weights are updated using the learning rate and gradient descent to reduce error.
-
Iteration: The process repeats until the model achieves the desired accuracy.
Challenges in Weight Optimization
-
Vanishing and Exploding Gradients: If weights are too small or too large, they can disrupt training.
-
Local Minima: Some weight adjustments can trap the model in suboptimal solutions.
-
Overfitting: Excessively tuned weights may cause the model to memorize training data instead of generalizing.
-
Computational Cost: Large-scale models with millions of weights require extensive computational power to optimize.
Techniques to Optimize Weights
-
Adaptive Learning Rates: Methods like Adam and RMSProp adjust learning rates to optimize weight updates efficiently.
-
Regularization: Techniques like L1/L2 regularization prevent excessive weight magnitudes, reducing overfitting.
-
Batch Normalization: Normalizing inputs before each layer helps stabilize weight distributions.
-
Weight Initialization: Strategies like Xavier or He initialization ensure proper weight distribution at the start of training.
-
Pruning and Quantization: Removing insignificant weights and compressing the model enhances efficiency without significant accuracy loss.
Some YouTube Videos to help explain AI Weights
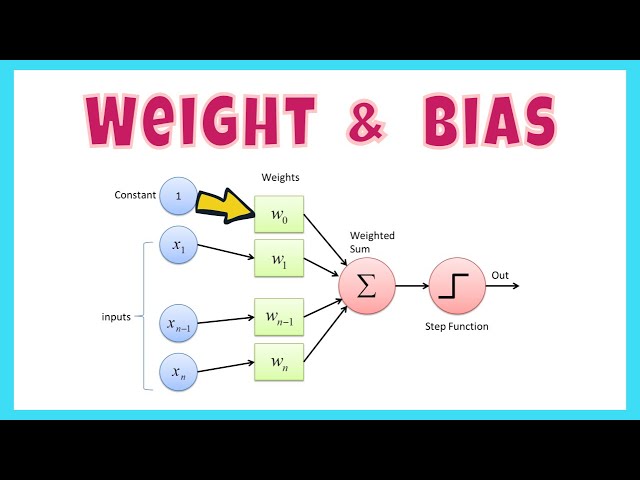
🔴 Weights and Biases in Neural Networks Explained in JUST 5 Minutes!
YouTube Channel: Analytics Camp
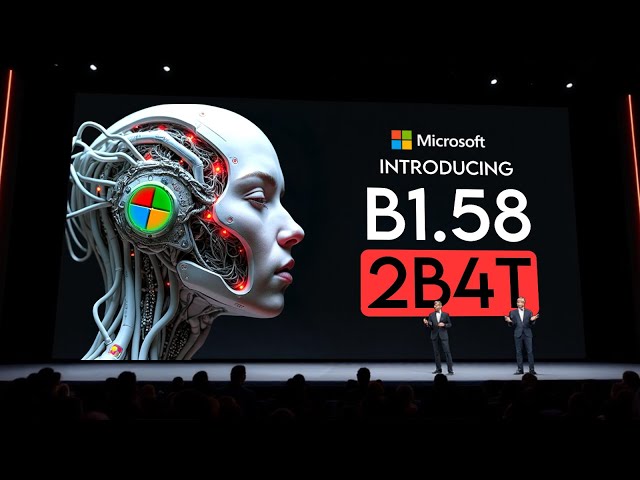
Microsoft Accidentally Created the Most Efficient AI Ever
YouTube Channel: AI Revolution
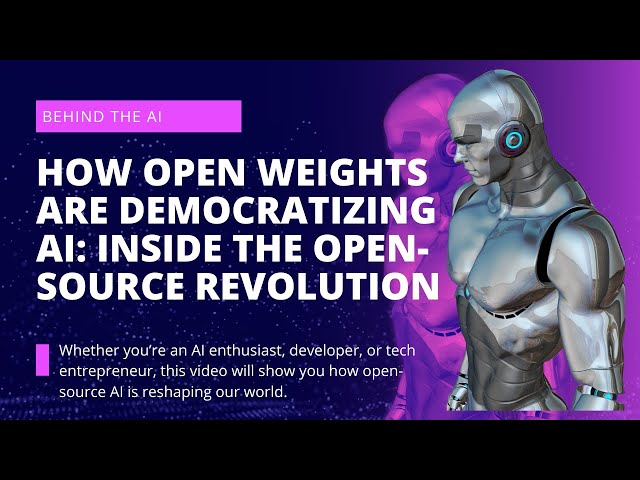
How Open Weights Are Democratizing AI: Inside the Open-Source Revolution
YouTube Channel: LifeOnAutomate
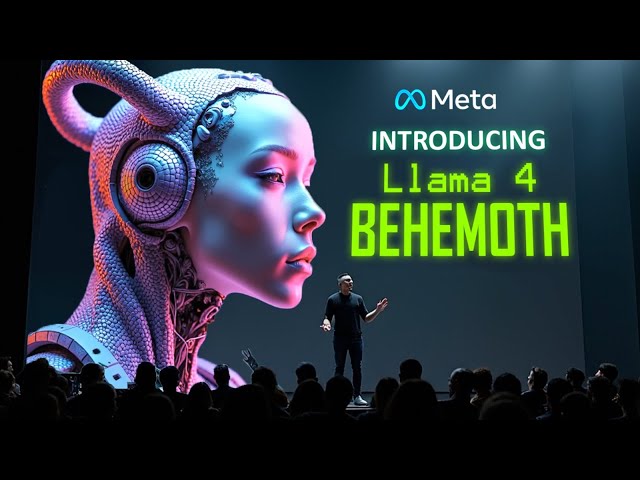
New 2 Trillion Parameter AI Model Shocks The World (Meta's Llama 4 Behemoth)
YouTube Channel: AI Revolution
Conclusion
AI weights are the core determinants of how machine learning models interpret and process data. Their careful optimization leads to better model accuracy, efficiency, and reliability. Understanding how weights function allows researchers and engineers to build AI systems that perform well across diverse applications, from image recognition to natural language processing.
By leveraging advanced optimization techniques, AI models can be fine-tuned to operate efficiently, making AI-driven solutions more effective and practical in real-world scenarios.
Trending AI Articles
IT and computer science courses
AI Questions and Answers section for What are AI Weights: The Backbone of Machine Learning Models
Welcome to a new feature where you can interact with our AI called Jeannie. You can ask her anything relating to this article. If this feature is available, you should see a small genie lamp in the bottom right of the page. Click on the lamp to start a chat or view the following questions that Jeannie has answered relating to What are AI Weights: The Backbone of Machine Learning Models.
Visitor asked: please explain in more detail what a Neural Network Activation weight is
Jeannie’s answer to the question: In machine learning and particularly in deep learning, weights play an integral role in modelling the significance of input features relative to the output. Specifically, in the context of a neural network, AI weights - more commonly referred to as "weights" - learn and decide how much influence or importance a particular input feature should have on the final output or prediction. These weights are used in the computation of every node in the hidden layers of a neural network. They influence the value that a neuron passes forward in the network during the activation function phase. Essentially, each input to a neuron is multiplied by a corresponding weight, which determines the contribution of the input. The result is then passed through an activation function, such as the ReLU or Sigmoid, that maps the input to the output. During the AI model training phase, these weights are fine-tuned through a process called backpropagation that uses techniques such as gradient descent to iteratively improve the model by reducing the error difference between predicted and actual outputs. The optimal weight values can drastically improve the accuracy and performance of AI models. Therefore, understanding and effectively adjusting neural network weights is a critical part of developing and training robust machine learning models.
Date: 2025-02-09
Visitor asked: In model complexity what are classed as excessive weights
Jeannie’s answer to the question: Excessive weights in model complexity are the weights in a machine learning or deep learning model that contribute to overfitting. These are weights that have been adjusted too specifically to the training data during the AI model training process. This extreme fine tuning can cause the model to perform poorly on unseen data because it's too intricately fit to the patterns and noise in the training set. Therefore, these excessive weights make the model less generalized, hindering its learning and predictive capabilities on new data. This is a common challenge when training complex models like deep neural networks. It's important to strike a balance in training to avoid weights that are too excessive or too limited, as both can negatively impact the model's performance.
Date: 2025-02-09
Visitor asked: Please elaborate on Techniques like L1/L2 regularization to optimize weights
Jeannie’s answer to the question: In machine learning models, particularly neural networks utilized in deep learning, AI weights play a critical role. They are numerical parameters that help the model in making predictions by strengthening or weakening the influence of input variables. The optimization of these weights is critical to the development of a strong and effective AI model. A key method for doing this involves using techniques such as L1 and L2 regularization. L1 and L2 are regularization techniques that add a penalty equivalent to the absolute value of the magnitude of coefficients (L1, also called Lasso regression) or the square of the magnitude of coefficients (L2, also called Ridge regression) to the loss function. This penalty, also known as a regularization term, discourages learning a more complex or flexible model, so as to avoid the risk of overfitting. L1 regularization has the propensity to shrink some parameters to zero, effectively excluding certain features entirely. This is especially useful when you suspect that only a subset of your features are actually influential. On the other hand, L2 regularization doesn't push for sparse solutions as much as L1 does, but rather promotes smaller and more distributed weight values. Both regularization techniques are used to prevent overfitting, which is a crucial challenge in machine learning. Overfitting happens when a model learns the training data too well, capturing noise along with inherent relationships. This typically results in poor performance on unseen, test data. By incorporating these techniques into AI model training, developers are able to create models that generalize better to unseen data and are thus more useful in practical AI applications.
Date: 2025-02-12