What Is Generative AI?
Publish Date: Last Updated: 17th April 2025
Author: nick smith - With the help of CHATGTP
Generative AI refers to a category of artificial intelligence algorithms that can create new content by learning patterns from existing data. Unlike traditional AI models that focus on recognizing patterns and making predictions, generative AI models produce original data that resembles the training data. This means they can generate text, images, music, and more that are novel yet coherent and contextually relevant.
At its core, generative AI employs machine learning techniques, particularly deep learning models like Generative Adversarial Networks (GANs) and Variational Autoencoders (VAEs). These models learn the underlying structure of the input data and use this understanding to generate new content that shares similar characteristics.
IT and computer science courses
How Does Generative AI Work?
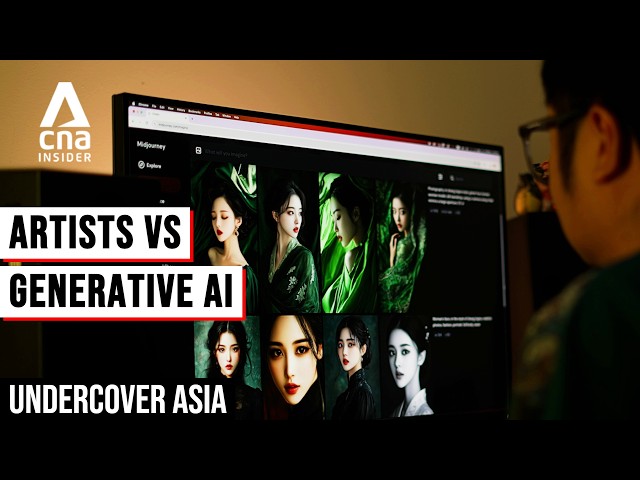
Copy + Paste + Steal: Artists Battle For Copyright vs Generative AI | Undercover Asia | Full Episode
YouTube Channel: CNA Insider
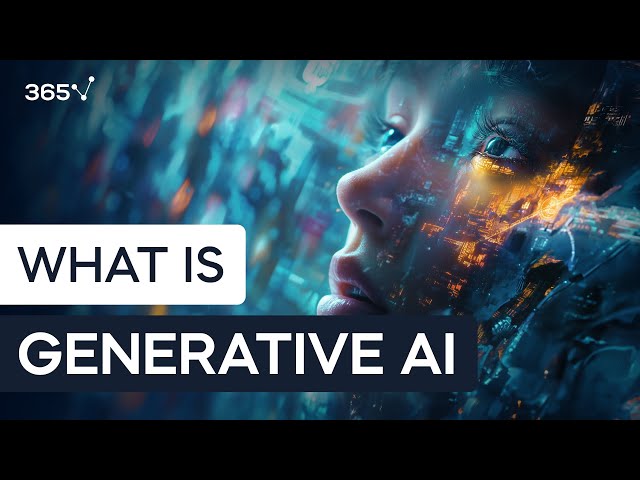
What Is Generative AI?
YouTube Channel: 365 Data Science
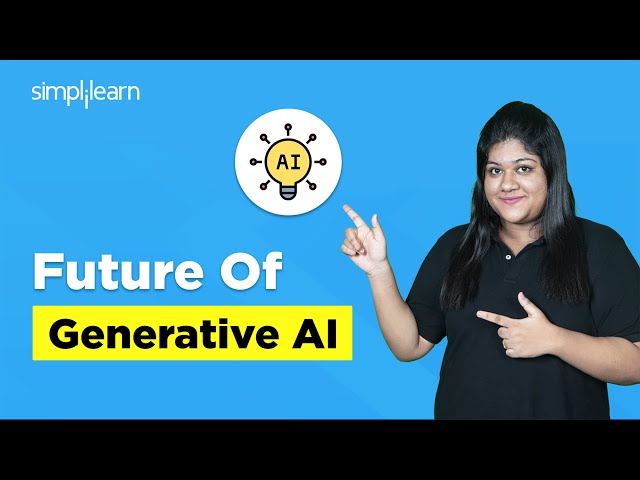
Future Of Generative AI | What Is Generative AI | Gen AI Advancement | Gen AI Explained |Simplilearn
YouTube Channel: Simplilearn
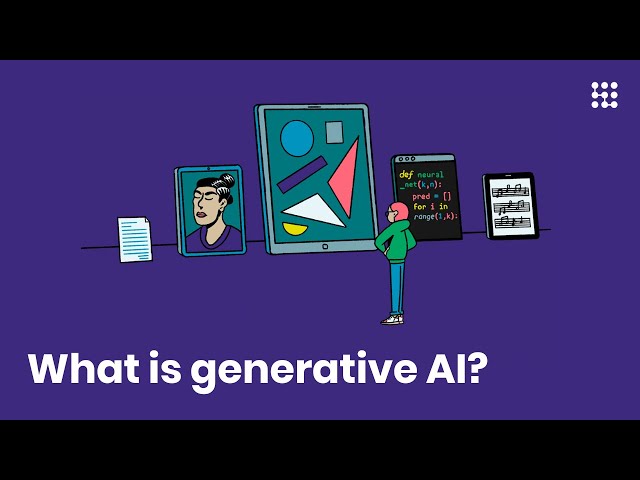
Generative AI explained in 2 minutes
YouTube Channel: KI-Campus
Generative AI models learn from large datasets to understand the data distribution and generate new instances. Here are two primary architectures used in generative AI:
1. Generative Adversarial Networks (GANs)
Introduced by Ian Goodfellow in 2014, GANs consist of two neural networks—the Generator and the Discriminator—that are trained simultaneously:
- Generator: Creates new data instances.
- Discriminator: Evaluates the generated data against real data.
The generator aims to produce data indistinguishable from real data, while the discriminator tries to identify whether the input is real or generated. Through this adversarial process, the generator improves over time, producing increasingly realistic data.
2. Variational Autoencoders (VAEs)
VAEs are another class of generative models that encode input data into a latent space and then decode it back to reconstruct the data:
- Encoder: Compresses the input data into a lower-dimensional latent representation.
- Decoder: Reconstructs the data from the latent space.
VAEs learn the distribution of the data and can sample from the latent space to generate new, similar data instances.
Applications of Generative AI
Generative AI has a wide array of applications across various industries:
1. Text Generation
- Chatbots and Virtual Assistants: Generative AI powers conversational agents that can understand and respond to human language naturally.
- Content Creation: AI models like GPT-4 can generate articles, summaries, and even creative writing pieces.
- Translation Services: Enhances machine translation by providing more contextually accurate translations.
2. Image Generation
- Art and Design: Tools like DALL·E 2 create original artwork based on textual descriptions.
- Fashion Industry: Generates new clothing designs by learning from existing styles.
- Architecture: Assists in creating building designs and layouts.
3. Music and Audio
- Composition: AI models can compose original music pieces in various genres.
- Sound Design: Generates sound effects for movies and games.
- Voice Synthesis: Creates realistic synthetic voices for virtual assistants and audiobooks.
4. Video and Animation
- Deepfakes: Alters videos to swap faces or modify speech, raising both creative opportunities and ethical concerns.
- Animation: Generates character movements and scenes in animated films.
- Gaming: Creates dynamic game content and environments.
Examples of Generative AI Tools
1. OpenAI's GPT-4
GPT-4 is a state-of-the-art language model capable of understanding and generating human-like text. It is used in various applications, including drafting emails, writing code, and creating content.
Example Usage:
- Content Generation: Writing articles, blog posts, and social media content.
- Programming Assistance: Generating code snippets or explaining code functionalities.
2. DALL·E 2
Developed by OpenAI, DALL·E 2 generates images from textual descriptions, allowing users to create unique visuals by simply describing what they want to see.
Example Usage:
- Design Prototyping: Visualizing product concepts before production.
- Creative Arts: Generating illustrations for stories or marketing materials.
3. DeepArt
DeepArt uses neural networks to apply the style of one image to another, transforming photographs into artworks resembling famous paintings.
Example Usage:
- Photography: Enhancing images with artistic styles.
- Marketing: Creating visually appealing graphics for advertising.
4. Jukebox
OpenAI's Jukebox generates music in various genres and styles, complete with lyrics and vocals.
Example Usage:
- Music Production: Composing original tracks for media projects.
- Entertainment: Creating custom music for games and virtual experiences.
The Future of Generative AI
The potential of generative AI is vast, with ongoing research and development expanding its capabilities:
- Personalization: Enhanced customization in products and services, tailored to individual preferences.
- Healthcare: Generating synthetic data for research while preserving patient privacy.
- Education: Creating personalized learning materials and interactive educational content.
- Ethical Considerations: Addressing concerns related to deepfakes, misinformation, and intellectual property rights.
As generative AI continues to evolve, it will play an increasingly integral role in innovation across sectors, driving efficiency and enabling new forms of expression.
Conclusion
Generative AI represents a significant leap forward in artificial intelligence, offering tools that not only analyze and predict but also create. By understanding and harnessing generative AI, businesses and individuals can unlock new levels of creativity and efficiency. Whether it's crafting compelling content, designing novel products, or composing original music, the applications of generative AI are limited only by our imagination.
Discover the endless possibilities with Generative AI and stay ahead in the ever-changing world of technology.
Trending AI Articles
AI Questions and Answers section for What Is Generative AI?
Welcome to a new feature where you can interact with our AI called Jeannie. You can ask her anything relating to this article. If this feature is available, you should see a small genie lamp in the bottom right of the page. Click on the lamp to start a chat or view the following questions that Jeannie has answered relating to What Is Generative AI?.
Be the first to ask our Jeannie AI a question about this article
Look for the gold latern at the bottom right of your screen and click on it to enable Jeannie AI Chat.