AI’s Environmental Impact
Addressing the Carbon Footprint of AI Models
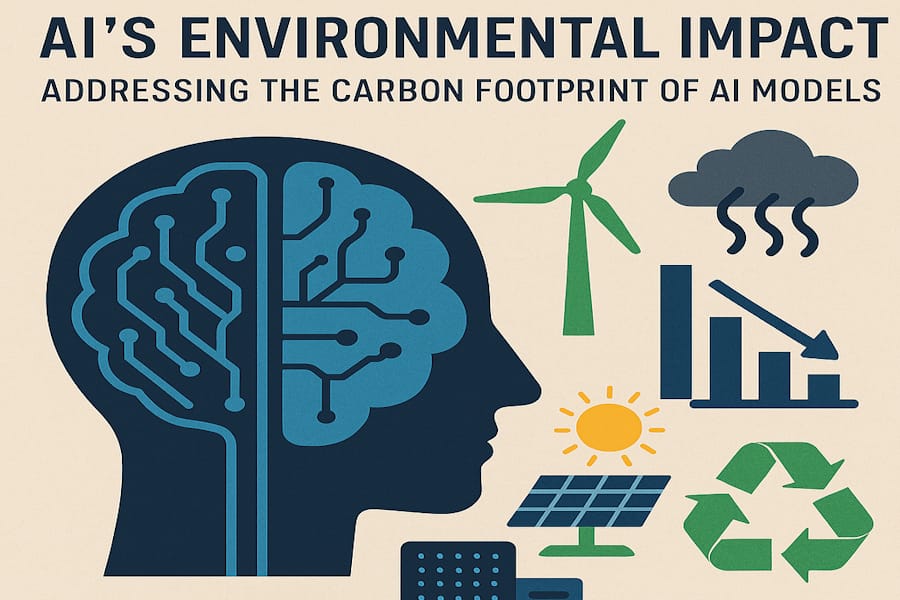
Publish Date: Last Updated: 16th May 2025
Author: nick smith - With the help of CHATGTP
Table of contents:
- Chapter 1 - Addressing the problem.
- Chapter 2 - A Green Distrabuted AI.
- Chapter 3 - Brain-Inspired AI: Neuromorphic Computing.
- Summary So Far: Rethinking the Energy Cost.
- Chapter 4 - Data-Efficient AI.
- Chapter 5 - AI for Energy Optimization.
Chapter 1
Artificial Intelligence (AI) has revolutionized various sectors, from healthcare to finance. However, this rapid advancement comes with significant environmental concerns, primarily due to the substantial energy consumption of AI models. As AI continues to integrate into our daily lives, it's imperative to understand and address its carbon footprint.
Develop your coding skills with an online course from FutureLearn. Join for free.
The Current Energy Landscape of AI
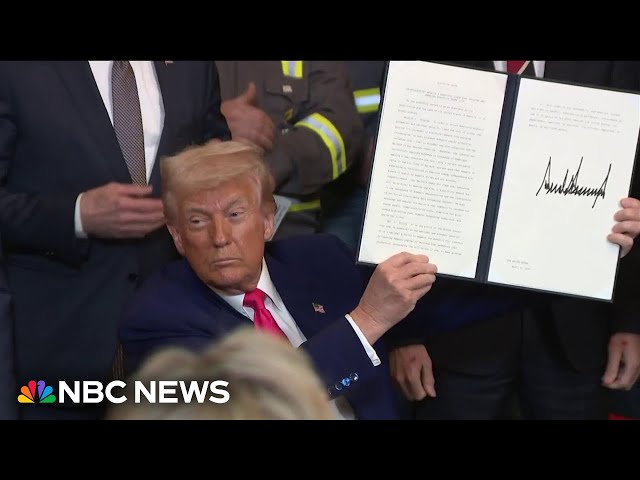
White House cites AI energy needs as reason for coal production boost
YouTube Channel: NBC News
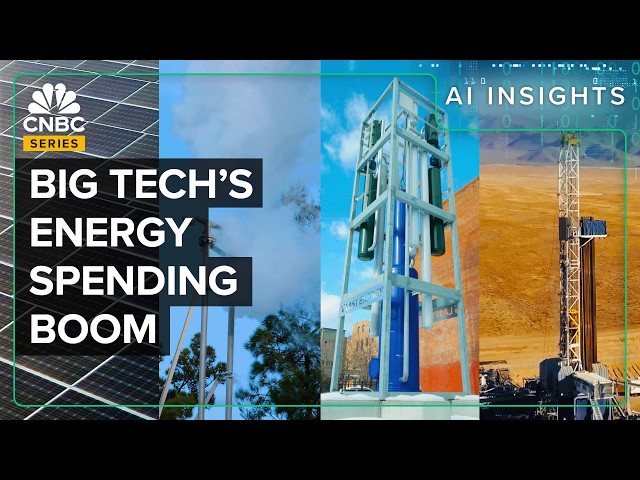
How Google, Microsoft And Amazon Are Racing To Solve The AI Energy Crisis
YouTube Channel: CNBC
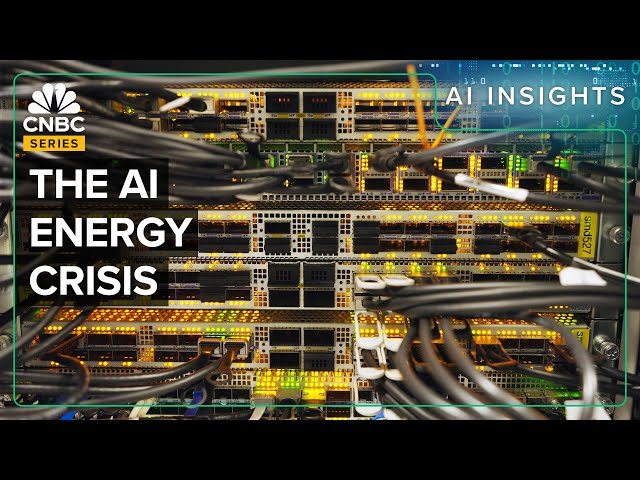
How The Massive Power Draw Of Generative AI Is Overtaxing Our Grid
YouTube Channel: CNBC
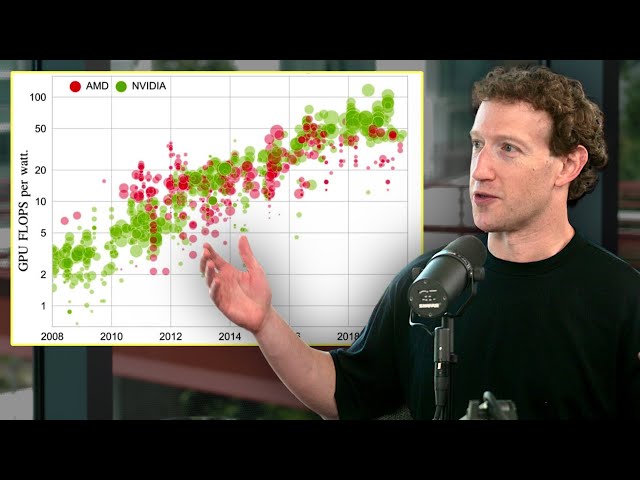
Energy, not compute, will be the #1 bottleneck to AI progress – Mark Zuckerberg
YouTube Channel: Dwarkesh Patel
Data Centers: The Powerhouses of AI
AI models, especially large-scale ones like GPT-3 and GPT-4, require immense computational resources. Training these models involves processing vast amounts of data, leading to significant energy consumption. For instance, training GPT-3 consumed approximately 1,287 megawatt-hours (MWh) of electricity, equivalent to the annual consumption of about 130 U.S. homes .Vox+1Home | Contrary+1
The proliferation of AI has led to a surge in data center construction. These centers are energy-intensive, with some consuming as much electricity as 100,000 households. By 2030, AI-focused data centers are projected to consume more power than Japan does currently .Wikipedia+1Latest news & breaking headlines+1Axios+1WSJ+1WSJ
Energy Sources Fueling AI
The energy powering AI data centers comes from a mix of sources:WSJ
-
Fossil Fuels: Despite a global push for cleaner energy, fossil fuels still play a significant role. In the U.S., coal contributes about 15% of the energy mix. Recent policies have even promoted coal to meet AI's growing energy demands .The Verge
-
Renewables: Solar and wind energy are increasingly being adopted. However, their intermittent nature poses challenges in meeting the constant energy demands of AI operations.
-
Nuclear Power: Some tech giants are turning to nuclear energy. For example, Microsoft has entered agreements to power its data centers with nuclear energy, aiming for a more stable and carbon-neutral energy source .Wikipedia
⚡ The Energy Cost of AI: Key Statistics
As AI technologies advance, their energy requirements have surged, leading to significant environmental concerns. Understanding the scale of energy consumption is crucial for developing sustainable solutions.
🔢 Key Energy Consumption Figures
-
Training Large AI Models: Training models like GPT-3 consumed approximately 1,287 megawatt-hours (MWh) of electricity, equivalent to the annual consumption of about 130 U.S. homes. Statista
-
Inference Energy Use: Each ChatGPT query consumes about 2.9 watt-hours, nearly 10 times that of a typical Google search. Statista+1Time+1
-
Data Center Consumption: AI-specific data centers are projected to quadruple their electricity consumption by 2030, potentially consuming more power than entire countries like Japan. WSJ
-
Global Impact: By 2030, data centers could account for up to 21% of global electricity demand when AI delivery costs are included. MIT Sloan
📊 Comparative Energy Consumption Table
Activity | Energy Consumption | Equivalent Comparison |
---|---|---|
Training GPT-3 | ~1,287 MWh | Annual consumption of ~130 U.S. homes |
Single ChatGPT Query | ~2.9 Wh | 10x energy of a standard Google search |
AI Data Centers (Projected 2030) |
945 TWh/year |
More than Japan's current annual electricity usage |
Global Data Center Electricity Demand (2030) | Up to 21% | Significant share of global electricity consumption |
Note: 1 MWh (megawatt-hour) = 1,000 kWh; 1 Wh (watt-hour) = 0.001 kWh
These figures underscore the pressing need for innovative solutions to mitigate AI's environmental impact. In the following chapters, we will explore potential strategies, including decentralized AI systems and energy-efficient computing architectures, to address these challenges.
Strategies for Sustainable AI
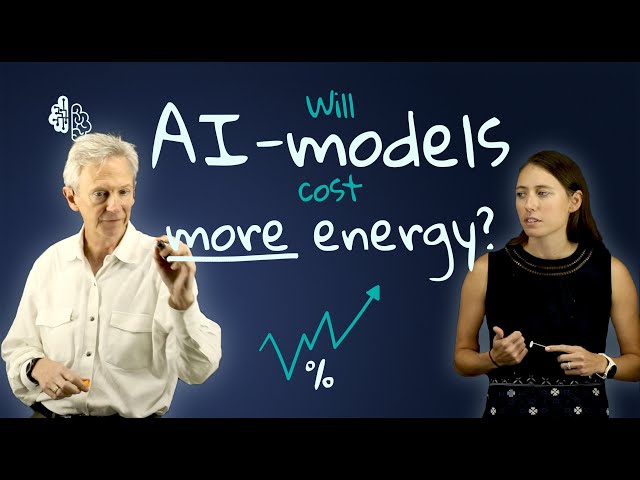
Is AI Sustainable? Exploring Its Energy Demands
YouTube Channel: Don Woodlock
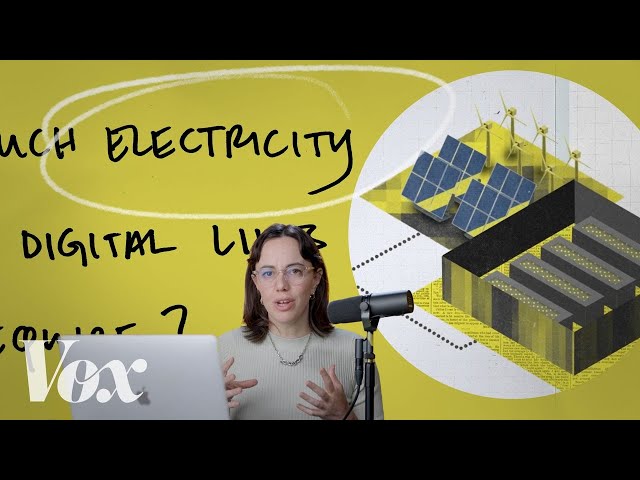
Can clean energy handle the AI boom?
YouTube Channel: Vox
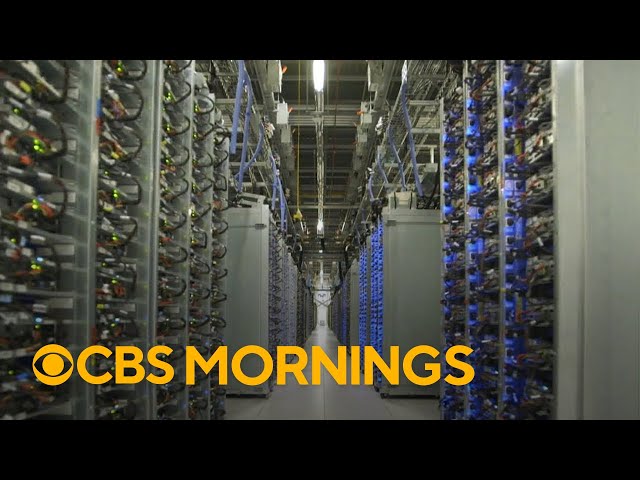
How AI and data centers impact climate change
YouTube Channel: CBS Mornings
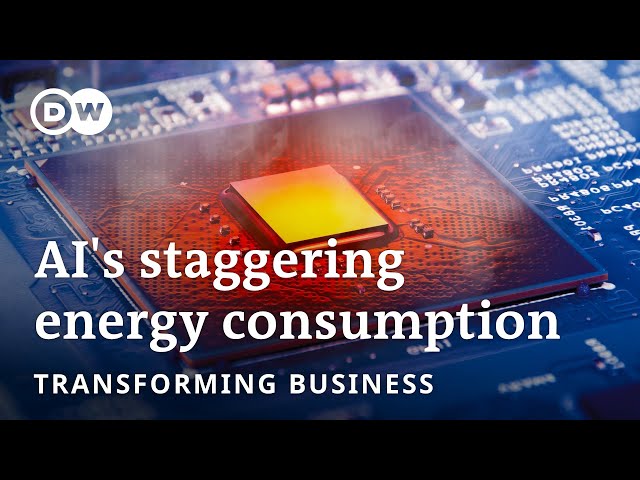
How AI causes serious environmental problems (but might also provide solutions) | DW Business
YouTube Channel: DW News
Enhancing Energy Efficiency
Reducing AI's energy consumption isn't solely about changing energy sources; it's also about making AI models more efficient:
-
Optimized Algorithms: Researchers are developing algorithms that require less computational power without compromising performance. For instance, certain techniques have reduced the energy required to train AI models by up to 80% .MIT News
-
Data-Centric Approaches: Focusing on the quality rather than the quantity of data can lead to significant energy savings. Modifying datasets has shown potential in reducing energy consumption by up to 92% without affecting accuracy .arXiv
Hardware Innovations
Advancements in hardware can lead to more energy-efficient AI operations:
-
Neural Processing Units (NPUs): These specialized chips are designed to handle AI tasks more efficiently than traditional CPUs or GPUs. NPUs can significantly reduce energy consumption and latency .Lifewire
-
Neuromorphic Computing: Inspired by the human brain, neuromorphic chips like Intel's Loihi aim to process information more efficiently, potentially reducing energy usage by up to 1,000 times compared to conventional systems .Wikipedia
Leveraging AI for Energy Management
Interestingly, AI can also be part of the solution:
-
Smart Energy Grids: AI can optimize energy distribution, reducing waste and improving efficiency.Pecan AI
-
Building Management: AI-driven systems can monitor and adjust energy usage in real-time, leading to significant savings in commercial buildings .
Conclusion
While AI offers numerous benefits, its environmental impact cannot be overlooked. By focusing on energy-efficient algorithms, innovative hardware, and sustainable energy sources, we can mitigate AI's carbon footprint. Moreover, leveraging AI itself to optimize energy usage presents a promising avenue. As we continue to integrate AI into various facets of life, prioritizing sustainability will ensure that technological advancement doesn't come at the expense of our planet.
🔍 From Problem to Possibility: AIBusinessHelp's Mission
At AIBusinessHelp, we believe that progress isn’t just about identifying what’s wrong—
it's about discovering innovative solutions that move us toward a smarter, more sustainable future.
While much has been said about the environmental cost of AI, we’re shifting the focus to something more hopeful:
How AI itself—and our collective intelligence—can be part of the solution.
The next chapter introduces a novel approach that combines community, creativity, and computation.
It’s a vision for improving AI performance while dramatically reducing the energy footprint of how it’s built and deployed.
This isn’t science fiction. It’s a scalable, decentralized strategy that could harness the idle power of millions of devices worldwide—just like humanity once united to search the stars.
Chapter 2
🌿 A Green Distributed AI: Crowdsourcing Intelligence for a Sustainable Future
As we search for solutions to reduce AI’s massive energy demands, we often look to better hardware, cleaner energy, or smarter algorithms. But what if the key to low-impact AI was already in your hands—literally?
Just like SETI@home once used idle home computers to search for extraterrestrial signals, we could harness millions (or billions) of everyday devices to power a decentralized, low-carbon AI ecosystem.
🔄 How Would It Work?
-
Users opt in to a global AI project by installing a lightweight client on their computers, tablets, or phones.
-
When the device is idle (e.g., at night), it runs micro-tasks that help train or optimize AI models.
-
Results are securely sent to a central (or decentralized) network, where they’re aggregated and used to improve global models.
This approach creates a massive, organic AI grid—distributed across the globe and powered by devices that would otherwise be idle.
💡 What Kind of Work Could Be Distributed?
-
✅ Inference tasks: Devices help process data (e.g., labeling, image classification, filtering).
-
✅ Model compression/pruning: Devices run optimization routines to reduce model size and energy needs.
-
✅ Federated learning: Models are trained locally on your device using your data, without ever leaving your system.
-
✅ Search space exploration: Crowd-participation in neural architecture search (AutoML).
-
✅ Synthetic data generation: Lightweight simulations to create new training examples.
🌍 Environmental Benefits
Traditional AI | Green Distributed AI |
---|---|
Massive centralized data centers | Millions of low-power devices |
Heavy GPU power usage | Energy-efficient CPUs/NPUs |
Relies on fossil-heavy grids | Leverages solar, home batteries, low-demand hours |
Scales energy linearly | Scales via idle infrastructure already in use |
🔐 Privacy and Security Built In
Using federated learning principles, this model:
-
Keeps user data on-device
-
Only shares anonymized model updates
-
Uses encryption and differential privacy
This mirrors what’s already done by companies like Google (for keyboard personalization) and Apple (for health models).
⚙️ Technical Enablers
To make this work, we’ll need:
-
Lightweight AI clients (cross-platform: Android, Windows, iOS, Linux)
-
Smart schedulers (run only when plugged in/charging or solar surplus is available)
-
Distributed orchestration (to coordinate millions of nodes)
-
Blockchain or token-based incentives (optional—users could earn rewards for participation)
🚀 Why Now?
-
Billions of connected devices exist globally
-
Energy prices and emissions are rising
-
Public interest in sustainable tech is higher than ever
-
Advances in federated learning, compression, and neuromorphic computing make it feasible
✨ A Global Intelligence Grid
Imagine an AI ecosystem that:
-
Reduces dependence on massive GPU farms
-
Empowers people to contribute directly
-
Uses renewable energy from homes and communities
-
Democratizes AI development across the planet
It’s not just possible—it’s the natural evolution of ethical, sustainable AI.
Chapter 3
🧠 Brain-Inspired AI: Neuromorphic Computing and the Path to Ultra-Efficient Intelligence
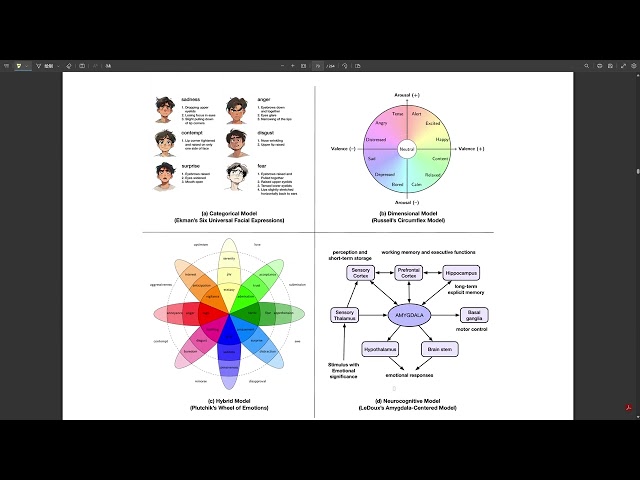
Advances and Challenges in Foundation Agents: From Brain-Inspired Intelligence to Evolutionary,
YouTube Channel: Xiaol.x
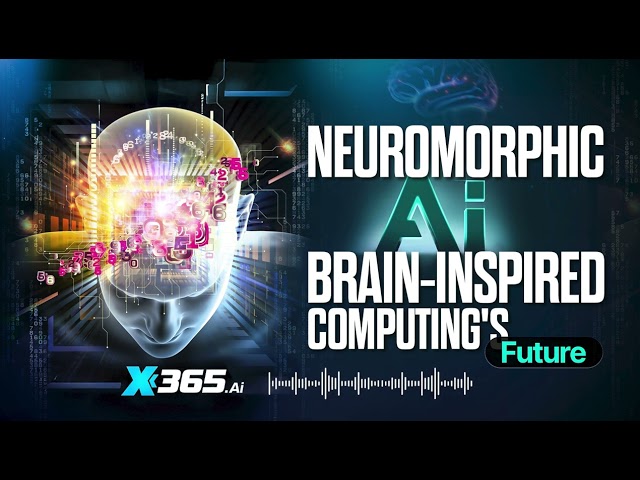
Neuromorphic AI: The Future of Brain-Inspired Computing!
YouTube Channel: X365
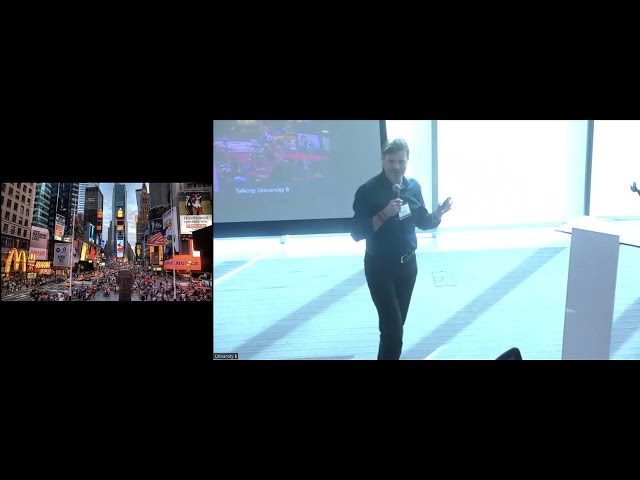
David J. Freedman, "AI-Inspired Neuroscience and Brain-Inspired AI"
YouTube Channel: UChicago Data Science Institute
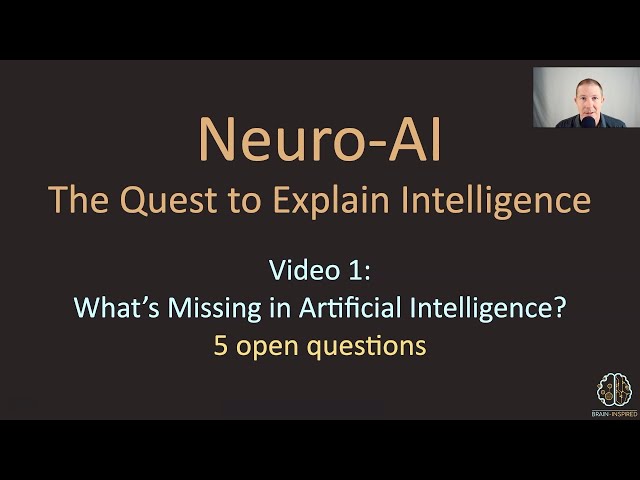
What's Missing in AI?
YouTube Channel: Brain Inspired
If you’re looking for the ultimate model of energy-efficient intelligence, you don’t need to look far.
It’s already in your head.
The human brain consumes about 20 watts of power—less than a light bulb—and yet it handles real-time pattern recognition, decision-making, learning, and multi-modal integration at a scale modern AI systems can’t match.
So why not take inspiration from nature?
That’s the idea behind neuromorphic computing—a radical approach that mimics the structure and function of biological neural networks, with the goal of creating machines that are not only smart but ultra energy-efficient.
🧬 What Is Neuromorphic Computing?
Neuromorphic computing involves designing processors that simulate the way neurons and synapses operate in the brain. Unlike traditional processors (CPUs/GPUs), which process data sequentially, neuromorphic chips use asynchronous, event-driven architectures to mimic the brain’s parallel, low-power computation.
Key principles:
-
Spiking neural networks (SNNs): Neurons “fire” only when needed, conserving energy.
-
Memory close to compute: Like the brain, memory and processing are tightly coupled.
-
Massively parallel: Thousands or millions of cores work simultaneously, like cortical columns.
🔬 Neuromorphic Hardware in Action
Neuromorphic Chip | Developer | Key Feature | Power Efficiency |
---|---|---|---|
Loihi 2 | Intel | On-chip learning and spiking neural support | 1000x more efficient than CPUs for specific tasks |
Tianjic | Tsinghua University | Hybrid classical and neuromorphic cores | Bridging symbolic + neural AI |
TrueNorth | IBM | 1 million neurons, 256M synapses | Consumes <100mW |
🧠 Intel’s Loihi chip can complete AI tasks using 100 to 1000 times less energy than traditional hardware—especially in tasks like voice recognition or sensory processing.
⚡ Why It Matters for Green AI
Neuromorphic systems don’t just reduce power use—they redefine the energy-performance equation.
Benefits include:
-
📉 Drastically lower energy consumption
-
🚀 Near real-time processing for edge devices
-
🔌 Minimal infrastructure requirements
-
📡 Ideal for always-on AI (e.g. wearables, IoT)
-
🌱 Scalable without exponential power growth
These systems could make AI truly deployable in remote or resource-limited environments, from smart agriculture sensors to planetary rovers—and all without needing megawatt data centers.
🧪 Use Cases Already Emerging
-
Smart prosthetics: Responsive, low-power interfaces controlled by neural signals.
-
Edge vision systems: Drones and cameras that “see” using micro-watts of power.
-
AI hearing aids: Real-time auditory processing with long battery life.
-
Neural implants: Bio-integrated chips mimicking brain activity.
🚧 Challenges and the Road Ahead
While promising, neuromorphic computing isn’t mainstream yet due to:
-
Limited software ecosystems (few tools/libraries like PyTorch/TensorFlow for SNNs)
-
Complexity of programming event-driven systems
-
Need for new AI models designed for SNNs instead of traditional ANNs
However, with rising pressure to green AI infrastructure, momentum is growing—and hybrid systems (combining neuromorphic + classical AI) are already in development.
🌍 A Brain-Inspired Path to Sustainable AI
Neuromorphic computing doesn’t just offer a smaller footprint—it offers a fundamentally different way to compute. By aligning AI’s future with the most efficient processor evolution has ever created—the human brain—we may finally unlock intelligence that scales with grace, not energy bills.
📘 Summary So Far: Rethinking the Energy Cost of Intelligence
As we've explored in the chapters above, artificial intelligence isn't just reshaping the world—it's also reshaping our energy demands.
AI’s carbon footprint is real and growing:
-
Training a single large model like GPT-3 can consume over 1,200 MWh—enough to power 130 homes for a year
-
Data centers are expected to quadruple energy usage by 2030, potentially consuming more electricity than entire nations
-
Even one ChatGPT request can use 10x the power of a Google search
But it's not just about identifying the problem. At AIBusinessHelp, it's about solutions—and we're already seeing incredible potential.
✅ Solutions We’ve Explored
🔋 Green Distributed AI
Inspired by SETI@home, this approach proposes using idle devices across the globe to power micro-tasks in AI development. It democratizes computation, reduces reliance on mega data centers, and could be fueled by solar and home energy networks.
🧠 Neuromorphic Computing
By mimicking the human brain, neuromorphic chips offer 100–1000x energy efficiency over traditional processors. They’re already powering low-energy AI in robotics, edge devices, and smart wearables—and may be the future of truly scalable AI.
These aren’t just ideas—they're working examples of a cleaner AI future already emerging.
🌱 What's Next?
Even with new hardware and distributed strategies, there’s one more frontier we must explore:
Reducing the sheer amount of data and computation we use in the first place.
Up next, we’ll dive into Data-Efficient AI—the art of doing more with less by rethinking how we train, optimize, and use models without wasting energy on redundant or low-quality information.
Chapter 4
🧮 Data-Efficient AI: Doing More with Less
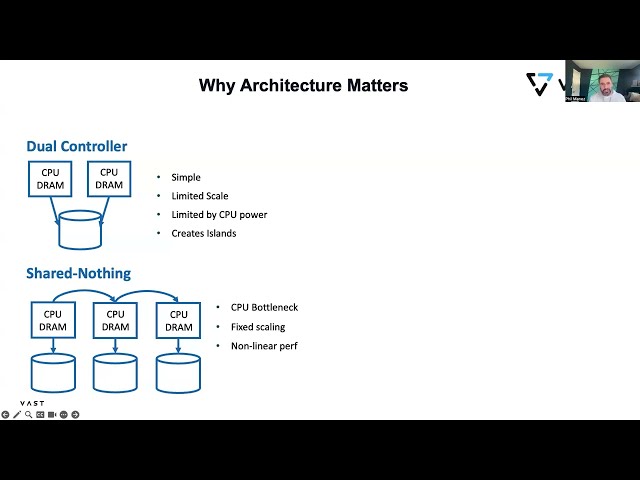
AI & Data Efficiency: The Power of Disaggregated Shared Everything Architecture
YouTube Channel: VAST Data
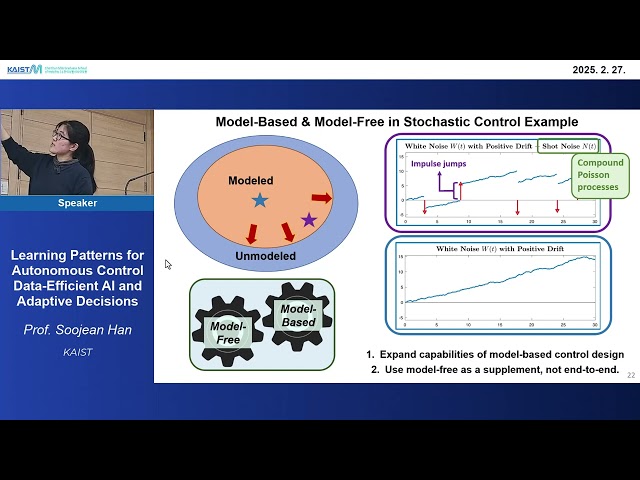
Learning Patterns for Autonomous Control Data Efficient AI and Adaptive Decision
YouTube Channel: KAIST ChoChunSik Graduate School of Mobility
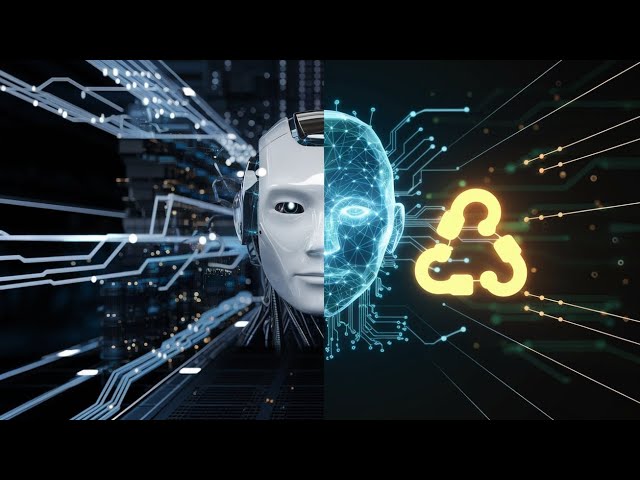
Open Source AI Rivals DeepSeek: Less Data, More Performance
YouTube Channel: Kim Shmy podcast
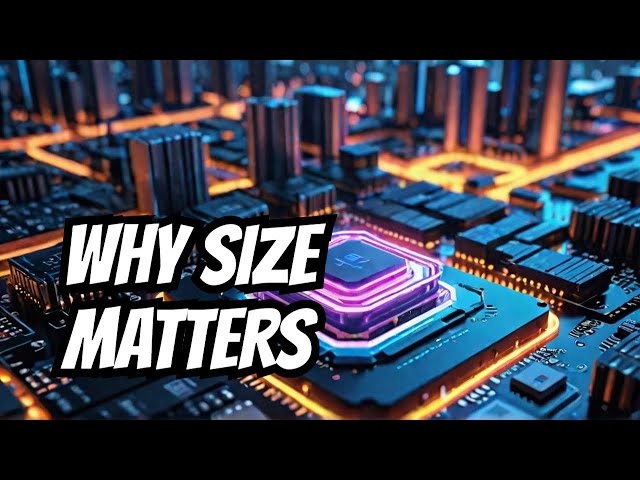
Why Smaller AI Models Are the Future!
YouTube Channel: AI Within Reach
If energy-efficient hardware is the body of green AI, then data efficiency is the brain’s discipline—the strategy behind making every bit of training count.
Modern AI models thrive on massive datasets and billions of parameters. But here’s the problem:
🔌 Bigger isn’t always better.
And more importantly — bigger burns more energy.
To build a truly sustainable AI future, we need to shift the mindset from brute force learning to smart learning.
🧠 The Hidden Waste of Overtraining
Every time we:
-
Train on low-quality or redundant data
-
Re-run expensive models for minimal gains
-
Add billions of parameters without better performance…
We’re not just wasting time.
We’re wasting megawatts of energy — and, by extension, carbon.
Studies have shown that up to 92% of training energy can be saved with smarter data selection strategies. (arxiv.org/abs/2204.02766)
💡 Techniques for Data-Efficient AI
1. 🧹 Data Curation Over Volume
Rather than feeding the model everything, we focus on clean, diverse, and relevant data.
-
Removes duplicates and noisy samples
-
Increases learning per sample
-
Reduces training time and energy
“Less, but better.” Think of it as AI on a minimalist diet.
2. 🎯 Curriculum Learning
Inspired by how humans learn—from easy to hard—this strategy:
-
Feeds simpler examples first
-
Gradually increases complexity
-
Leads to faster convergence with fewer iterations
💡 Result: Less compute. Faster results. Smaller carbon footprint.
3. 🔄 Transfer Learning & Pretrained Models
Instead of training from scratch, start with a model already trained on general data, and fine-tune it for your task.
✅ Saves up to 95% of training energy
✅ Reduces dataset size needs
✅ Makes AI more accessible to small teams
4. 🧠 Active Learning
Don’t label everything. Let the model ask for the most informative data points.
-
Focuses human effort and compute on what matters
-
Great for real-world use where labeling is expensive
5. 🗜️ Model Compression
Shrink the model after training with techniques like:
-
Quantization (e.g., from float32 to int8)
-
Pruning (removing unnecessary weights)
-
Distillation (teaching a smaller model using a larger one)
These can reduce model size by 50–90%, with minimal performance loss—massively reducing energy during inference.
⚡ Real-World Impact
Strategy | Energy Reduction | Bonus Benefit |
---|---|---|
Transfer learning | Up to 95% | Faster deployment |
Curriculum learning | ~50% | Smarter convergence |
Model pruning | 40–80% | Lighter model for devices |
Active learning | 60–90% (labeling) | Reduces human effort + data |
🔄 Smarter AI Is Greener AI
Building better AI doesn't mean building bigger AI.
It means building it intelligently—the way nature does: adaptively, efficiently, and with a deep respect for limited resources.
Chapter 5
♻️ AI for Energy Optimization: When AI Helps Power Itself, Smarter
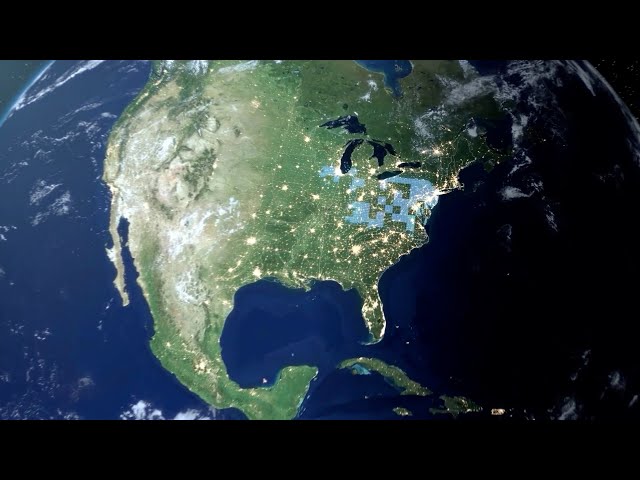
Investing in AI-Powered Solutions for the Electric Grid
YouTube Channel: Google
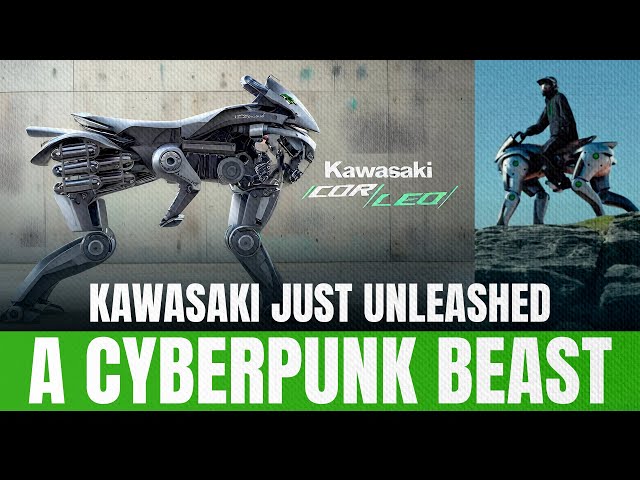
Meet CORLEO: Kawasaki’s Futuristic, Hydrogen-Powered AI Horse! | FrontPage
YouTube Channel: AIM TV
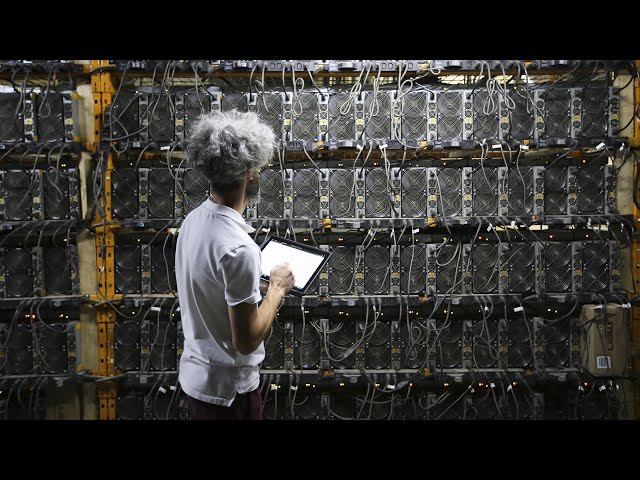
Demand for Next-Generation AI Data Centers
YouTube Channel: Bloomberg Television
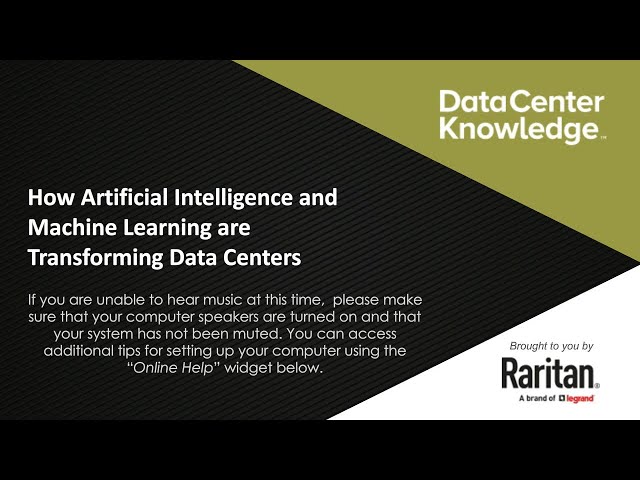
How Artificial Intelligence (AI) and Machine Learning are Transforming Data Centers (Webinar)
YouTube Channel: Raritan
We’ve explored how to reduce AI’s carbon footprint through distributed systems, brain-inspired hardware, and smarter data practices. But what if AI could actually help solve its own energy problem?
That’s not science fiction—it’s happening now.
By applying AI to the very systems that power our world, we can optimize energy use, eliminate waste, and build an infrastructure where AI isn’t just a consumer—but a contributor to sustainability.
🔌 Where AI Can Make a Difference
🏢 1. Smart Data Centers
Data centers are the engine rooms of modern AI—and also some of the biggest energy consumers.
AI is now being used to:
-
Optimize cooling systems and airflow
-
Predict and shift workloads to off-peak hours
-
Power down idle servers dynamically
Google uses DeepMind’s AI to manage cooling in its data centers—reducing energy usage by up to 40%.
🏙️ 2. Grid-Level Energy Management
AI helps balance and predict energy demands on national grids:
-
Forecast renewable energy supply (wind, solar)
-
Shift loads based on usage patterns
-
Prevent blackouts and reduce reliance on fossil backups
Think: AI systems running on AI-optimized grids. Full circle.
🧱 3. AI in Building Efficiency
In commercial buildings and campuses:
-
AI adjusts HVAC systems in real time
-
Predicts occupancy and adjusts lighting accordingly
-
Integrates local renewables with battery storage
One AI company reduced building energy usage by up to 30% using real-time predictive controls.
🚘 4. AI-Powered Transportation
Logistics, public transit, and EV routing all benefit from AI:
-
Optimized routes = less fuel and electricity used
-
Smarter charging = reduced strain on the grid
-
Autonomous systems = fewer emissions over time
🧠 5. Meta-Optimization: AI That Reduces Its Own Cost
Some of the most exciting research now looks at:
-
Dynamic model serving (only run heavy models when truly needed)
-
Task-aware inference (use simpler models for simpler tasks)
-
Energy-aware training schedules (based on cost and carbon intensity)
Imagine an AI system that asks: “Can I do this task with 10% of the energy?” — and then does it.
🌍 The Final Thought
Artificial intelligence is one of the most powerful tools humanity has ever created. But with great power comes a great electricity bill.
The good news?
AI doesn’t have to be part of the problem.
It can be part of the solution—if we build it that way.
From distributing computation across the world, to mimicking the brain, to minimizing data waste, to optimizing the energy systems it relies on…
We have the blueprint for a truly sustainable, intelligent future.
Now it's up to us—builders, researchers, decision-makers—to choose the smarter path forward.
Trending Articles
AI Questions and Answers section for AI Carbon Footprint Solutions
Welcome to a new feature where you can interact with our AI called Jeannie. You can ask her anything relating to this article. If this feature is available, you should see a small genie lamp in the bottom right of the page. Click on the lamp to start a chat or view the following questions that Jeannie has answered relating to AI Carbon Footprint Solutions.
Be the first to ask our Jeannie AI a question about this article
Look for the gold latern at the bottom right of your screen and click on it to enable Jeannie AI Chat.